Maximize Your Organizational Success with a Data Centric Approach
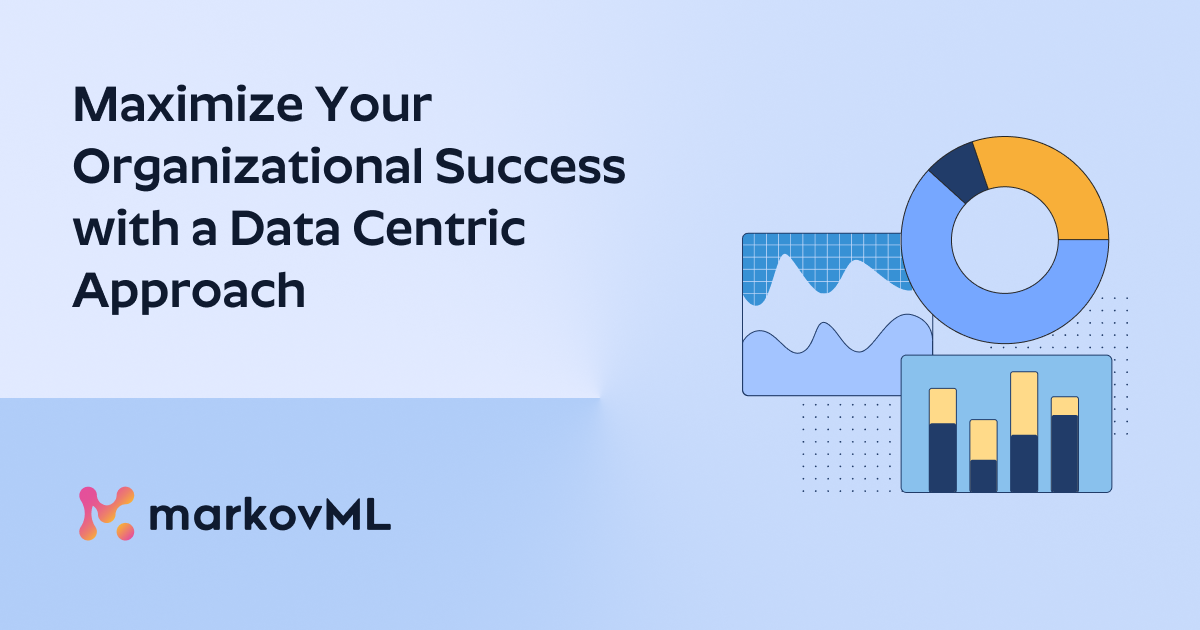
The transformative impact of a data-centric approach on organizational success is undeniable.
According to the McKinsey Global Institute, companies that embrace data-driven strategies are 23 times more likely to outperform in customer acquisition, six times more adept at retaining customers, and 19 times more likely to achieve profitability.
This underscores the immense power of a data-centric strategy, making it a critical focus for businesses aiming to thrive in an increasingly competitive landscape. In this blog, we will discuss the know-how of building truly data-centric organizations.
Understanding Data-Centric Approach
A data-centric approach implies that strategic or operational decisions are guided by data insights rather than intuition.
A Forrester study involving 300 senior executives revealed that business leaders recognize the necessity of a data-centric ethos to stay ahead of customer expectations and market opportunities.
In fact, 62% of business owners say that data centricity and analytics give them a competitive advantage. But the benefits aren’t limited to just that; there are plenty. Let's have a look.
Benefits of a Data-Centric Approach in Organizations

1. Enhanced Decision Making
Organizations can make more informed, evidence-based decisions with a data-centric approach. This leads to improved efficiency and effectiveness in operations.
2. Customer Insights
By leveraging data, businesses gain a deeper understanding of customer behavior and preferences, facilitating personalized experiences.
3. Risk Management
Data-centric strategies aid in identifying and mitigating risks by analyzing trends and patterns.
4. Innovation and Competitiveness
Access to and using quality data drives innovation, keeping organizations competitive in their respective industries.
5. Operational Optimization
Data-centric ML and computing enable the automation and optimization of various processes, leading to cost savings and increased productivity.
What are the Key Elements of a Data-Centric Approach
A data-centric approach is systematic and principled towards data excellence by improving data quality throughout the lifecycle of a machine learning project. It involves emphasizing its strategic value in driving decision-making instead of merely considering it an incidental by-product of business operations.
Here are some key elements of a data-centric approach:
1. Data Management
Essential for a data-centric approach, this involves meticulous preparation, lifecycle management, and version tracking of high-quality, relevant data.
2 .Automation & Pipelines
Crucial in scaling AI projects, it focuses on automating data annotation and management workflows, including pre and post-processing datasets.
3. Data Quality
A core element deeply integrated with data engineering, enhancing data reliability and transparency for more accurate AI models.
4. Data-Centric Security
Prioritizes data security at every stage—collection, storage, and usage—to protect against loss and detect potential threats.
5. Hybrid Approach
Balances the focus on data and models, adopting a strategy aligning with specific project needs and outcomes.
How to Implement a Data-Centric Approach
A successful data-centric approach requires a clear strategic roadmap, robust governance, and a supportive infrastructure. Here's how organizations can effectively implement this approach:
1. Defining Data Strategy and Objectives
The first step is to define a clear data strategy aligned with the organization's broader goals. For instance, a retail company might use customer data to personalize shopping experiences, while a healthcare provider might prioritize patient data to improve treatment outcomes.
This strategy should outline how data will be collected, analyzed, and utilized to drive decision-making processes.
2. Data Governance and Management
Once the strategy is established, establishing strong data governance is essential. This includes setting policies for data access, quality, privacy, and security.
For example, a financial institution might implement stringent data governance policies to ensure compliance with regulatory requirements while safeguarding sensitive customer information.
Effective data management also means having data storage, archiving, and retrieval systems, ensuring data is accessible and usable when needed.
3. Data Infrastructure and Tools
The backbone of a data-centric approach is robust data infrastructure and the right set of tools. This might involve investing in cloud storage solutions for scalability, employing data-centric machine learning platforms for advanced analytics, or adopting data-centric testing tools for quality assurance.
For example, a logistics company might use advanced analytics tools to optimize routes and reduce fuel consumption, leveraging data-centric architecture for real-time data processing and decision-making.
Data-Centric vs. Data-Driven
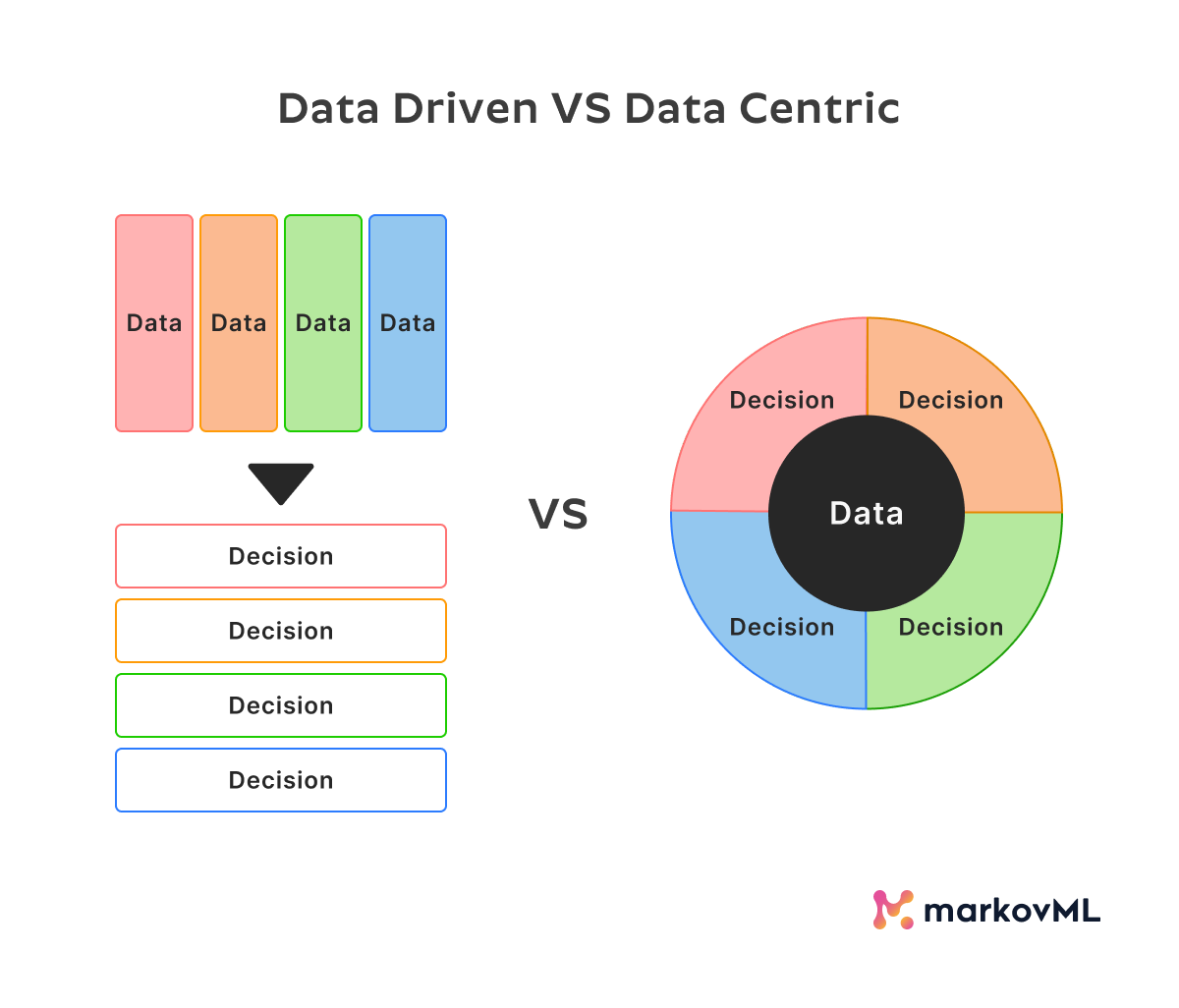
While often used interchangeably, 'data-centric' and 'data-driven' embody distinct organizational decision-making and strategy philosophies. Understanding their differences is pivotal for businesses harnessing the power of data effectively.
Here’s an elaborate differentiation between both; Data-Centric vs. Data-Driven approaches
Data-Centric vs. Model-Centric Approach
The choice between a data-centric and a model-centric approach can significantly influence the success of AI initiatives. Here's an elaborate distinction between both approaches:
Overcoming Challenges in Building a Data-Centric Approach
Building a data-centric approach in an organization can be a transformative process, but it comes with its own challenges. Overcoming these is key to leveraging the full potential of a data-centric methodology.
1. Data Security and Privacy Concerns
As organizations collect and utilize more data, ensuring its security and privacy becomes paramount. This involves deploying advanced cybersecurity measures and complying with data protection regulations like GDPR.
For instance, a financial institution must encrypt sensitive customer data and regularly update its security protocols to safeguard against breaches.
2. Data Quality and Integration
Ensuring the quality and integration of data from diverse sources is a significant challenge. Data quality can lead to accurate data analysis and decision-making. Organizations should implement robust data management systems to cleanse, standardize, and integrate data from various sources.
For example, a retail chain might use data integration tools to merge online and offline customer data for a unified view.
3. Organizational Culture and Change Management
Shifting to a data-centric approach often requires a culture change. Employees at all levels need to understand the value of data and be trained in data literacy. Change management strategies should be employed to foster a culture where data-driven decision-making becomes the norm.
A tech company, for instance, might hold workshops and training sessions to embed data-centricity in its corporate culture.
Best Practices for Successful Implementation
To successfully implement a data-centric approach in an organization, certain best practices are pivotal. These practices facilitate the transition and ensure long-term sustainability and effectiveness.
1. Building a Data-Centric Culture
The foundation of a data-centric approach is a culture that values data as a critical asset. This involves educating and training employees across all levels to appreciate and utilize data in decision-making.
For instance, a manufacturing company might conduct regular workshops to teach staff how to interpret data from production metrics and apply these insights to improve efficiency.
2. Continuous Learning and Improvement
A data-centric approach thrives on continual learning and evolution. Regularly updating data strategies and technologies in line with emerging trends is vital.
Implementing mechanisms for feedback and learning, such as A/B testing or data-centric machine learning models, ensures that strategies remain relevant and effective.
In the tech industry, this could mean continuously refining data algorithms based on user engagement data to enhance product offerings.
3. Measuring and Reporting Data-Driven Outcomes
To validate the effectiveness of a data-centric approach, it's crucial to have robust metrics and reporting mechanisms in place. These help quantify the impact of data-driven decisions and strategies, foster accountability, and guide future initiatives.
A healthcare provider, for example, could track improvements in patient outcomes or operational efficiency as key metrics to measure the success of their data-centric practices.
Embracing the Future with Data-Centric AI
Adopting a data-centric approach in organizations is not just about leveraging data for operational efficiency; it's about transforming data into a strategic asset that drives innovation and growth.
By focusing on data quality, integration, and a culture that embraces continuous improvement and data-driven outcomes, organizations can harness the full potential of their data resources.
Explore the world of data-focused AI and think about the role of platforms like Markov in this journey. It helps you speed up your journey from data to actionable intelligence, making raw data useful more quickly and efficiently.
Try out Markov and feel confident about moving forward into the world of data-focused AI.
Let’s Talk About What MarkovML
Can Do for Your Business
Boost your Data to AI journey with MarkovML today!