Artificial Intelligence (AI) has made incredible progress in the past few years, with adoption rates increasing each year. As per McKinsey’s State of AI in 2022 report, the average number of AI capabilities used in an organization doubled from 1.9 in 2018 to 3.8 in 2022.
However, while the usage of AI is increasing, it is also a cause of concern for most organizations, especially in the context of data governance challenges.
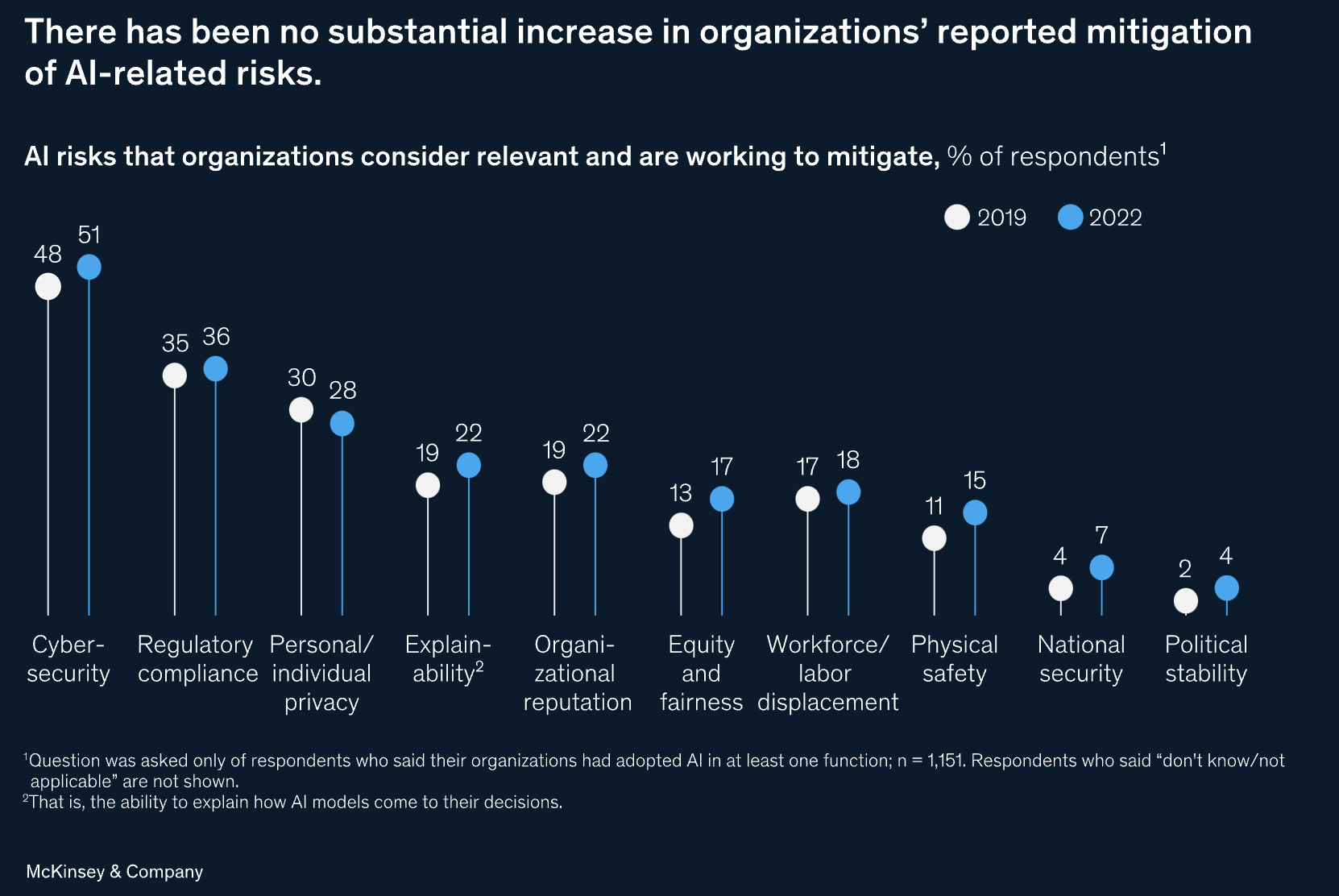
This blog will dig deeper into the data governance challenges in AI, the collaborative efforts required, and the data governance future trends you should be aware of. Understanding these challenges is essential for harnessing the full potential of artificial intelligence.
Understanding Data Governance Challenges in AI
Data governance in AI focuses on outlining various policies or procedures that ensure data integrity, quality, security, and usability. It plays a pivotal role in ensuring data's ethical and responsible use.
Data privacy and governance are a cause for concern as AI proliferates in various industries and use cases. For example, if the data fed to AI for fraud detection includes personal classifiers, these can inadvertently get used for reporting or visualization, compromising the privacy of that individual.
Using Data Governance protocols, businesses can help encrypt or protect this data from any external usage and ensure that it complies with regulatory frameworks and laws.
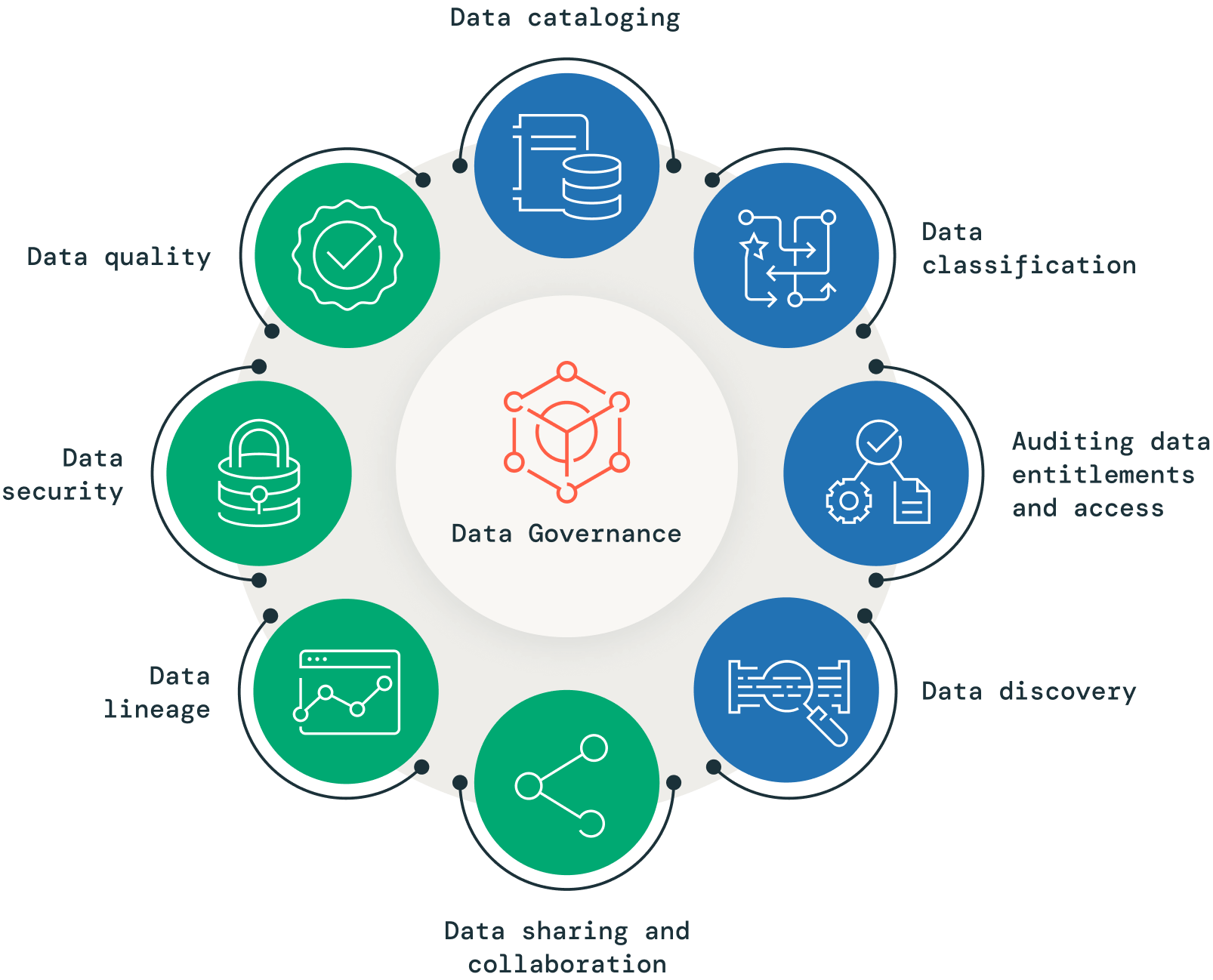
Some of the data governance challenges that organizations face when implementing AI solutions are as follows:
1. Data Privacy
AI models can often process sensitive data, such as names, bank account details, transaction details, and more, for processes such as fraud detection. This raises concerns about data privacy and the potential for misuse since AI cannot differentiate between private and public data.
Organizations must implement robust data privacy measures, such as data anonymization and access controls, to ensure that sensitive information is always protected.
2. Data Quality
The quality of data used to train AI models directly affects the accuracy and reliability of those AI models. Data governance practices should focus on identifying and correcting data errors, inconsistencies, and missing values.
For example, data that is outdated or inconsistent can affect the accuracy and fairness of AI models. To ensure that this doesn't happen, organizations need to enforce clear data quality standards, which ensures that error-prone or inconsistent data is not used for model training.
3. Resource Constraints
Implementing and maintaining effective data governance in AI often requires significant resources in terms of personnel, technology, and expertise. For example, in the case of fraud detection mechanisms, organizations need resources that have both technology and domain expertise. This right combination ensures that the personnel can train the AI model to detect fraud and know which results are positive and which are false positives.
Organizations must allocate adequate resources to make sure that their data governance program can meet the demands of AI initiatives.
4. Data Security
AI systems are vulnerable to cyberattacks, making data security a critical concern. Data governance frameworks must incorporate robust security measures, such as encryption and privacy controls, to protect data from unauthorized access or disclosure.
5. Compliance and Regulatory Issues
Many regulations govern the collection, use, and disclosure of data. For example, if you are collecting private details like phone numbers, email, and others for an upcoming event, the customer should be provided with options to regulate how this information is used. If this is not done, companies can face heavy fines as per local regulations.
Organizations must ensure that their data governance implementation complies with all applicable regulations, such as the General Data Protection Regulation (GDPR) in the European Union.
Overcoming Data Governance Challenges
Data governance challenges may continue to play a part, especially when effectively implementing and maintaining AI models. To overcome these data governance challenges in AI, organizations can implement a few strategies, such as:
a. Data Privacy and Compliance
The key to data governance starts with robust practices to protect data privacy and ensure compliance with regulations. This involves creating transparent data usage policies, implementing access controls, and conducting regular audits to verify compliance.
To implement these processes, organizations should follow a few of the below steps:
- Define privacy policies and principles: Clearly define data privacy policies and principles, including guidelines and data usage protocols, to ensure compliance with data privacy regulations.
- Protect sensitive data: Implement technologies like data encryption, data access controls, and other protocols to protect sensitive data and information from being utilized or leaked.
- Mask private information: AI models can also be trained to mask private information before usage to ensure it doesn’t inadvertently make it into analysis and reporting.
- Regular data audits: Regularly audit data holdings for compliance with privacy regulations and identify potential risks or breaches promptly.
b. Data Quality and Integration
To ensure clean and validated data for AI models, organizations must enhance data quality and integration by following a few steps as shown below:
- Data Quality Controls: Implementing data quality standards and establishing data validation mechanisms to cleanse data errors.
- Centralized Data Repository: Establishing a centralized data repository to consolidate data from disparate sources, ensuring consistency and accessibility.
- Master Database Management: Implementing MDM solutions to manage key master data entities and have a uniform reporting format, improving data consistency and accuracy.
c. Data Security
The biggest challenge with AI models is the inadvertent usage and the leak of sensitive information. To protect data from sensitive information, organizations should:
- Access Controls: Implement robust access control mechanisms to restrict unauthorized access to sensitive data, including user authorization, role-based access, and multi-factor authentication.
- Data Encryption: Utilize encryption techniques, both in transit and at rest, to safeguard data during transmission and storage.
- Regulatory Training: Conduct training and awareness programs for their teams to maintain data privacy and mitigate associated risks.
- Network Monitoring: Deploy IDS/IPS solutions to monitor network traffic and identify potential cyberattacks, enabling timely remediation.
d. Compliance and Regulatory Issues
Addressing compliance and regulatory issues forms the crux of any data governance exercise. Noncompliance can not just lead to fines or legal challenges but can also break users' trust.
To stay protected, organizations should:
- Update Data Governance Policies: Review and update data governance policies and procedures to align with evolving data privacy and security regulations.
- Access Risk Protocols: Regularly assess data risks associated with data breaches, unauthorized access, and data misuse to prioritize mitigation efforts.
- Data Breach Protocols: Develop comprehensive data breach response plans to effectively handle data breaches, minimize damage, and comply with regulatory requirements.
Benefits of Collaborative Data Governance
Collaborative data governance is an approach that puts the onus of managing data governance protocols on every team member. This ensures cooperation and coordination among stakeholders, ensuring that data usability, integrity, and security protocols get implemented throughout the process.
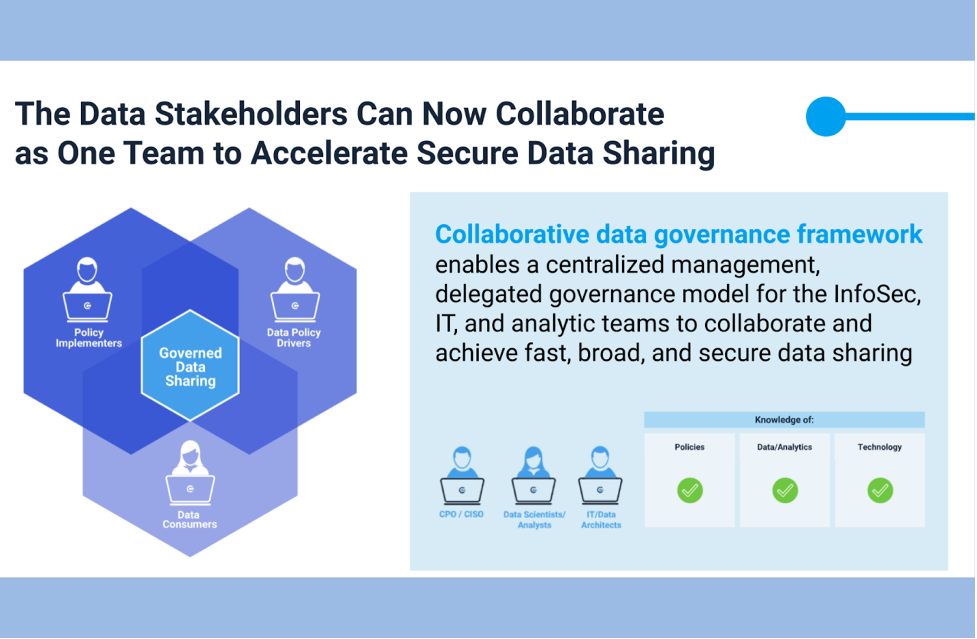
This collaborative approach can yield several notable benefits:
- Enhanced Data Quality: By pooling expertise and resources, cross-functional teams can identify and address data quality issues more effectively, leading to cleaner, more consistent data for AI models.
- Improved Data Security: Data governance collaboration promotes open communication and transparency, proactively identifying and mitigating potential data security risks. This shared responsibility instills a culture of data security throughout the organization.
- Efficient Resource Allocation: Having each resource working on data governance protocols streamlines data management processes, reducing duplication and ensuring that resources are allocated effectively. This efficiency frees up time and resources for more strategic initiatives like AI development and innovation.
- Streamlined Compliance: By involving key stakeholders in data governance decisions, organizations can ensure that their data practices align with applicable regulations and industry best practices. This collaboration helps minimize compliance risks and potential penalties.
- Accelerated Innovation in AI Development: Collaborative data governance fosters a culture of knowledge sharing and collaboration, enabling teams to access and utilize data more effectively. This shared access to high-quality data accelerates AI development and innovation.
The Future of Data Governance in AI Development
As AI is used in more processes and evolves further, data governance will play an increasingly critical role in ensuring the responsible and ethical development of AI systems. For ethical use of data for training ML models, future business practices need to focus on the following:
- Data Capturing and Classification, ensuring that every data that is captured in the system is properly categorized, enabling AI to know which data it can use for training and which to avoid
- Embed controls to enforce policies and regulatory best practices for data and AI
- Continuous validation through statistical analysis and the use of AI to ensure that data quality is always consistent and meets organizational standards
Conclusion
Data governance will be key to building trust and transparency in AI development, enabling organizations to leverage AI effectively while upholding ethical principles and safeguarding data privacy. By addressing data privacy, quality, security, resource allocation, and compliance challenges, organizations can truly unlock the full potential of AI.
With AI and ML models evolving rapidly, organizations must manage advanced model complexities and implementation challenges to provide a structured governance framework. MarkovML's Data Intelligence features can help you effortlessly speed up data analysis, evaluation, and data organization for faster results. It comes with intelligent features, such as:
- Collaborative Reporting
- Intelligent Data Cataloging using AI workflows for seamless discovery, traceability, and lineage
- Reusable and automated templates to automate manual tasks, improving data sharing, management, and governance.
Platforms like MarkovML can help organizations implement deep data insights and automated workflows and collaborate seamlessly with other tools. This helps to create a framework for responsible and impactful AI development, which will be crucial in the future.
FAQs
1. What is data governance in the context of AI?
Data governance in AI refers to a set of policies, practices, and processes that ensure data for AI development is managed, protected, and used ethically. It encompasses guidelines for ensuring optimal data quality, privacy, security, and compliance.
2. Why is data governance important in AI development?
Data governance in AI development is crucial for ensuring that the data used to train and operate AI models is accurate, unbiased, and compliant with applicable regulations. Without adequate data governance implementation, the usage of this data is undefined and can lead to misuse or even changes in data.
3. What are the common data governance challenges in AI?
Common data governance challenges in AI include data privacy concerns, data quality issues, resource constraints, data security risks, and compliance with regulatory requirements.
4. What is the future of data governance in AI development?
As more organizations and businesses use AI, the need for data to be protected and properly utilized will only increase. Thus, organizations must adopt comprehensive data governance frameworks that prioritize data quality, security, and compliance to harness the power of AI while minimizing potential risks.