Exploring Data Profiling Tools and Their Benefits
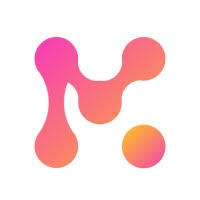
The amount of data being created, captured, and consumed globally is increasing rapidly and is projected to reach 180 zettabytes by 2025. With rising data inflow comes the need to properly store, manage, and analyze this data, making data analytics a crucial element.
It is thus no wonder that the big data analytics market is projected to reach $68 billion by 2025, with data profiling being the most critical factor for business success.
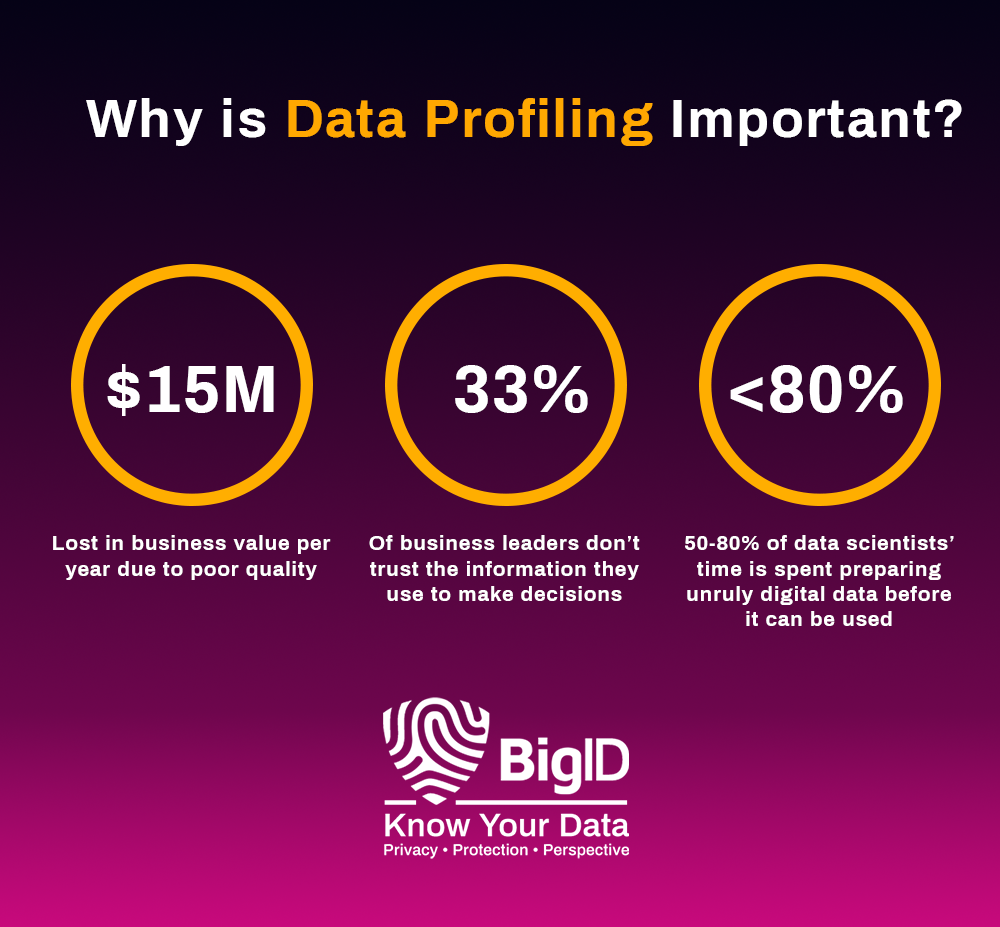
This article will delve into the role of data profiling tools in enhancing data quality, types of data profiling, and data profiling techniques that you should be aware of.
What are Data Profiling Tools?
Data profiling refers to the process of examining, analyzing, reviewing, and summarizing data sets to gain insight into the quality of data.
This process of automated data profiling is done using data profiling tools or software that are designed to access and analyze datasets, helping data professionals to understand their data better. It helps them make more informed decisions and ensure high data accuracy.

Database profiling typically follows a variety of techniques to analyze data, including:
- Data sampling: It may sample a subset of data to identify patterns and trends.
- Data type validation: Validates data types to ensure data is in the correct format.
- Data completeness and consistency checks: To ensure there is no missing or inconsistent data.
- Data relationship analysis: To identify relationships between different data sets.
Benefits of Using Data Profiling Tools
Data profiling tools are proven to help data professionals in more ways than one. They empower organizations to gain deeper insights into their data, leading to more informed decision-making and improved operational efficiency. This helps to improve organizational data processes in three ways:
1. Identification of Data Quality Issues
Data profiling helps to identify data quality issues, which include the following:
- Data fields that are missing or incomplete
- Inconsistent data fields, such as customer names spelled differently in different systems
- Invalid data fields, such as email addresses, that are not in the correct format
- Duplicate data records
- Outliers, such as customer sales that are significantly higher or lower than the average
By pinpointing these problems, organizations can proactively address data quality concerns, leading to more reliable analysis and reporting.
MarkovML can help simplify data analysis and speed up data evaluation with simple clicks. With Auto Data Analyzers powered by AI, you can easily identify data gaps, outliers, and patterns to make informed modeling decisions without requiring you to write complex codes for deep insights.
2. Improved Data Accuracy
One of the primary benefits of fixing data quality issues is improved data accuracy. By systematically analyzing data and flagging inaccuracies, organizations can correct and cleanse their datasets, ensuring that the information they rely on is trustworthy and error-free
Thus, businesses can ensure that their data is more accurate and reliable. While this process required a lot of manual interventions, it can now be automated with - automatic relabeling workflows in MarkovML, reducing the time spent on fixing the data issues to a few clicks.
These workflows can boost team productivity by allowing you to efficiently build data workflows from scratch using an intuitive drag-and-drop interface. You may use pre-built templates or reuse your previous workflows to define a workflow, helping you save time by automating monotonous data tasks and eliminating the need for human intervention.
This, in turn, enables them to make data-driven decisions, improving their operational efficiency and developing new products that are in tune with data trends.
3. Efficient Data Cleansing and Transformation
Automated data profiling also helps businesses to cleanse and transform their data efficiently. Data profiling tools can automate many tasks involved in data cleansing and transformation. They provide insights into data dependencies and relationships, enabling organizations to perform necessary transformations with precision.
This can save businesses time and money and reduce the risk of human errors in the data preparation phase, further enhancing data integrity.
Common Features of Data Profiling Tools
Data profiling tools offer a range of common features that make them indispensable in data analysis. These features typically include data summarization, data structure analysis, and content assessment.
1. Data Profiling Metrics
Data profiling tools can be used to assess data against several metrics, including the following:
- Completeness: The percentage of data values that are present.
- Consistency: The degree to which data values are consistent across different systems and processes.
- Accuracy: The degree to which data values are correct.
- Uniqueness: The percentage of data values that are unique.
- Validity: The degree to which data values meet the expected format and range.
These metrics help organizations evaluate their database for issues or underlying challenges and take corrective actions.
2. Data Quality Reporting and Visualization
Data profiling tools help generate reports that summarize the results of data analysis. These reports can further identify data quality issues, track data quality trends over time, and communicate data quality findings to stakeholders.
This reporting and visualization functionality assists data professionals and stakeholders in understanding data quality at a glance and supports informed decision-making in data management and data-driven projects.
Types of Data Profiling
The data profiling process can typically be categorized in these three ways:
- Structured Discovery: Structure profiling analyzes the format and structure of data, including data types, column names, and data relationships (between tables).
- Content Discovery: Content profiling analyzes the content of data, such as the values of data fields, the distribution of data values, and the relationships between different data fields.
- Relationship Discovery: Relationship profiling analyzes the relationships between different data sets. This can help to identify inconsistent data, duplicate data, and outliers.
How to Choose the Right Data Profiling Tool
Consideration Factors
When choosing a data profiling tool, there are a number of factors to consider, including:
- The type of data to be profiled: Data profiling tools can be used to profile various data types, including structured data, unstructured data, and semi-structured data. It is essential to choose a tool that can profile the specific types of data.
- The size of the data: In addition to the type of data, you need to know the typical size of the data that will be profiled, from small data sets to large data lakes. The tool that you choose should be able to handle the size of the data that you need to profile.
- The complexity of the data profiling tasks to be performed: You need to determine what data features will be used, such as data discovery, data quality assessment, data enrichment, data visualization, and reporting.
- The budget: Data profiling tools can range in price from free to open source to commercial. It is important to choose a tool that fits your budget.
Leading Data Profiling Tools
If you are looking specifically at data profiling tools, you can choose from:
- Talend
- Informatica Data Quality & Observability
- IBM InfoSphere Information Analyzer
- Microsoft SQL Server Data Quality Services
Each tool offers unique features and capabilities to address various data profiling requirements.
In addition to these tools, you can consider MarkovML. It empowers data scientists and ML engineers to understand and prepare their data for machine learning projects, thereby contributing to data profiling and data quality improvement.
By providing insights into data, tracking model training experiments, evaluating models, and explaining outcomes, MarkovML accelerates the data preparation phase, leading to more accurate and reliable data for data profiling.
Data Profiling Examples
Data profiling can be implemented in several scenarios, especially ones where data quality is of utmost importance. Some of the data profiling examples include:
- Data Transformation projects, where data needs to be sanitized and cleansed to be usable. This step is crucial for creating efficient prediction models. Data profiling before any analysis helps to improve data quality and accuracy, making it important for any AI project.
- Other data proving usage includes troubleshooting data issues, especially when you have large and complex data. For instance, you can use a SAS data loader for Hadoop to identify and resolve issues within a database. This helps to improve data quality metrics and improve accuracy.
- Data profiling can also identify issues and corrections in extract, transform, and load (ETL) processes. This is crucial for data conversion or migration processes, as cleansing data before it is moved to another system helps with data quality challenges. Data profiling tools can also help to identify issues that may occur due to relocation or adaptation of data in new systems and help mitigate migration errors.
Conclusion
Data profiling is crucial in any organization and requires proper planning and execution. The most efficient way to manage the data profiling process is to automate it with data-driven solutions. By embracing these data profiling tools, organizations can unlock the full potential of their data, setting the stage for data-driven success in a rapidly evolving digital landscape.
In this quest to triumphantly manage data integrity and governance processes, you can bank on MarkovML. You can simplify data analysis, speed up data evaluation, and make organizational data management easier in just a few clicks.