Effective Deployment Strategies Machine Learning Models
The gap between development and deployment is a critical hurdle in machine learning. Recent research from D2iQ reveals that a staggering 87% of AI projects never reach production, highlighting the urgency of effective ML deployment strategies. This statistic is a wake-up call underscoring the importance of bridging this gap.
Imagine a retail company adopting an ML model to predict customer buying patterns, showing potential in a controlled environment. Transitioning to real-world deployment, however, poses challenges, including integrating the model with existing IT infrastructure and adapting it to align with their systems.
Besides, successful implementation requires cross-departmental collaboration, ensuring the model's compatibility and effective use in tailoring marketing strategies, personalizing customer experiences, and enhancing inventory management. Hence deployment becomes a crucial step, blending technical and operational integration.
This article aims to demystify the strategies needed for a successful deployment process.
Fundamental Strategies for ML Models
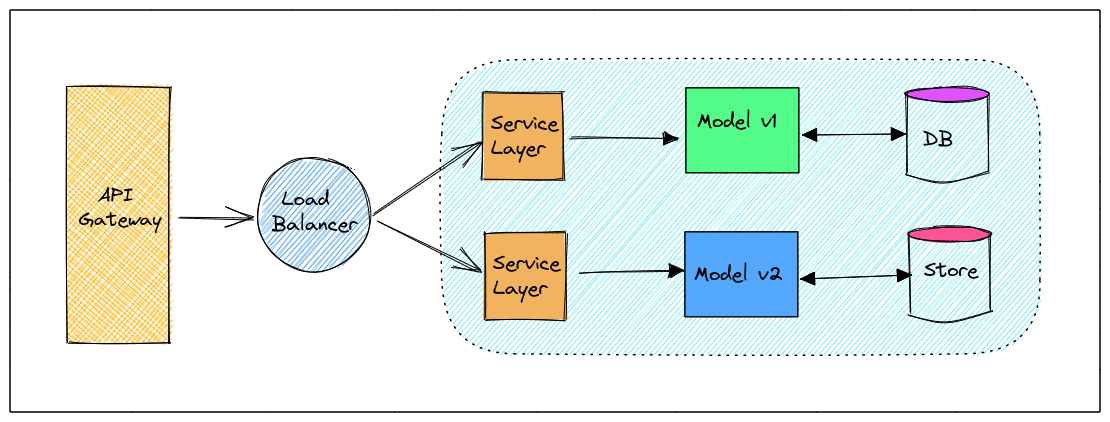
The significance of deploying machine learning models effectively is underscored by a recent IDC forecast, predicting that worldwide spending on AI systems will grow to nearly $154 billion by 2023. This growth is indicative of the increasing emphasis on not just developing, but successfully deploying AI solutions across various sectors.
1. Laying the Groundwork with Data Preprocessing
This phase is crucial for ensuring the model's readiness. Model evaluation involves rigorously testing the model against various scenarios to ensure its accuracy and reliability. For instance, a financial institution might evaluate its fraud detection model against historical fraud cases to gauge its effectiveness.
Data preprocessing, on the other hand, involves cleaning and organizing data to improve model performance. A healthcare provider, for example, might preprocess patient data to remove inconsistencies before deploying a disease prediction model.
2. Choose the Right Deployment Environment
This step determines where and how the model will operate. Cloud-based deployment, where the model is hosted on cloud services like AWS or Azure, offers scalability and flexibility. A retail chain, for example, might use cloud deployment for its demand forecasting model to easily scale during peak seasons.
On-premises deployment, where the model runs on local servers, provides greater control and security. A defense organization, for example, might prefer on-premises deployment for a sensitive reconnaissance image analysis model to ensure data security. Each environment has its merits and is chosen based on the specific needs of the application.
3. Keeping Track and Staying Alert: Model Versioning and Monitoring
Effective model deployment begins with version control, ensuring that changes and iterations are tracked meticulously. For instance, a financial forecasting model might undergo multiple revisions to improve accuracy, and versioning allows reverting to previous iterations if needed.
Continuous monitoring is also vital. Consider a healthcare diagnosis model; continuous monitoring can quickly identify and rectify any drift in accuracy due to evolving medical data patterns.
4. Mastering Scalability and Efficiency in ML
When handling large datasets, scalability is key. An e-commerce site might use an ML model to recommend products. As the product range and customer base grow, the model must scale accordingly without loss in performance.
Model optimization techniques, such as pruning and quantization, can enhance efficiency. For example, a traffic management system uses optimization to process data faster, ensuring real-time traffic predictions.
5. Making Interfaces Approachable and Integrative
Deployed models must feature user-friendly interfaces. A weather prediction model, for instance, should provide easy-to-understand forecasts for a wide range of users. Integration with existing systems is equally important for seamless operation.
In a manufacturing setting, integrating a predictive maintenance model with the existing machinery monitoring systems can streamline operations, making the model more accessible and actionable for plant technicians.
6. Security and Compliance in Model Deployment
In the realm of ML deployments, safeguarding data and adhering to regulations are paramount. Security measures, such as encryption and access controls, are critical for a healthcare company using ML to handle sensitive patient information.
Simultaneously, navigating the legal landscape is vital. For instance, a financial institution must ensure that its AI-driven credit scoring system adheres to financial regulations like GDPR or the Fair Credit Reporting Act to mitigate risks of non-compliance and maintain consumer trust.
7. Mastering Model Updates and Retraining
The dynamic nature of data demands that ML models evolve continuously. An e-commerce platform, by implementing incremental learning, can refine its recommendation algorithms based on the latest user interactions, enhancing personalization and user experience. Similarly, retraining is crucial for long-term accuracy and relevance.
A meteorological agency, for example, must frequently retrain its weather prediction models to incorporate new data patterns and environmental changes, ensuring the forecasts remain reliable and precise. These practices of updating and retraining are essential for the sustained effectiveness and relevance of ML models in a rapidly changing world.
Future Trends in Machine Learning Model Deployment

As we look towards the horizon of machine learning (ML) and artificial intelligence (AI), several emerging trends are set to redefine the landscape of ML model deployment.
These developments not only promise to enhance the efficiency and effectiveness of deployments but also aim to address the evolving challenges and opportunities in the field. Here are some key trends to watch:
- Automated Model Optimization: The future will see a rise in automated tools for optimizing ML models. These tools will intelligently fine-tune models for better performance and efficiency, reducing the need for manual intervention and accelerating the deployment process.
- Increased Emphasis on Ethical AI: As AI becomes more pervasive, there will be a heightened focus on developing and deploying ethically responsible models. This involves ensuring fairness, eliminating biases, and enhancing transparency in how models make decisions.
- Edge Computing Integration: The integration of edge computing in ML deployments will grow. By processing data closer to the source, edge computing reduces latency, enhances data privacy, and improves model responsiveness, especially in IoT applications.
- Hybrid Cloud Environments: Hybrid cloud strategies, combining private and public clouds, will become more prevalent. This approach offers flexibility, scalability, and enhanced security, allowing organizations to leverage the strengths of both environments for optimal model deployment.
- Cross-disciplinary Collaboration: The deployment of ML models will increasingly require collaboration across various fields – data science, IT, business strategy, and ethics. This interdisciplinary approach will be key in addressing complex challenges and unlocking the full potential of AI applications.
- Focus on Model Monitoring and Maintenance: Continuous monitoring and maintenance of deployed models will be essential to ensure they adapt to changing data and environments, maintaining accuracy and relevance over time.
- Quantum Computing Integration: As quantum computing matures, its integration with ML will revolutionize model capabilities, offering unprecedented processing power and solving complex problems faster.
Final Thoughts
In conclusion, the journey of deploying ML models is intricate yet vital, demanding strategies that encompass security, compliance, scalability, and continuous adaptation to technological advancements and data dynamics. This requires not just technical acumen but also a holistic approach that considers ethical AI, cross-disciplinary collaboration, and emergent technologies like quantum computing.
Hence platforms like MarkovML become invaluable. As a data-centric AI platform, MarkovML simplifies and streamlines these complex deployment processes, ensuring models are not only effectively integrated into operational workflows but also continuously optimized for evolving business needs.
Its focus on user-friendly interfaces and collaborative features positions it as an essential tool in the arsenal of any organization aiming to leverage the full potential of AI and ML technologies.
Let’s Talk About What MarkovML
Can Do for Your Business
Boost your Data to AI journey with MarkovML today!