The Morals of Progress: Mitigating Ethical Concerns in GenAI Systems

Deep neural networks and machine learning algorithms allow GenAI systems to learn from their input data and promise a greater future. According to a 2023 survey by Salesforce, 33% of surveyed IT leaders mention generative AI as a top priority.
However, apart from the technological promises, the concept of GenAI also raises many eyebrows due to concerns about its ethical use. These concerns serve as challenges to the ethical deployment and widespread use of generative AI systems.
In this blog, we will discuss the implications of these concerns and provide a comprehensive framework for responsible GenAI development, which can serve as a blueprint for mitigating the ethical concerns associated with generative AI systems.
Overview of Ethical Concerns

The multifaceted nature of GenAI systems makes the ethical concerns surrounding their use all the more complex. The ethical use of generative AI is essential for preventing its misuse and mitigating concerns around the security of sensitive data.
According to the same survey by Salesforce, 79% of IT leaders are concerned about the security risks surrounding generative AI. The rising adoption of GenAI raises the following concerns:
- Biases in GenAI algorithms
- Privacy issues due to GenAI's data-centric nature
- Lack of transparency
- The inability of laws to keep up with GenAI developments
Without proper consideration and resolution of these complex challenges, the benefits that GenAI systems offer will remain tainted by the risks associated with their deployment.
Significant Ethical Concerns in GenAI Systems
Before supporting conversations around mitigating concerns related to GenAI systems, it is imperative to gain a deeper understanding of the ethical conundrums plaguing the field, serving as a foundational layer of context for a dynamic situation.
Let's look further into the following specific challenges that need to be dealt with:
1. Bias in AI Algorithms
Bias in GenAI systems remains a persistent ethical concern, driven by their propensity to inherit and consequently worsen biases present in the data used for their training.
According to a 2023 Applause survey, 47% of respondents claimed to have received biased responses from generative AI systems. These biases can produce discriminatory outputs and lead to inequitable outcomes.
The adoption of more proactive measures during the training phase can help establish equally representative datasets, with proper monitoring of generative AI algorithms helping identify and deal with any present biases.
2. Privacy Issues
The robust deployment of GenAI systems requires large sets of user data for their continuous development and refinement. This data requirement raises concerns regarding the proper safeguarding of this data, especially in fields that deal with sensitive individual data, such as healthcare and finance.
According to the Applause survey, 67% of respondents felt that GenAI services tend to infringe on data privacy. Dynamic data encryption methods and strict privacy laws and regulations are vital for ensuring that sensitive data is protected while still allowing for its meaningful and transparent use in algorithm training.
3. Transparency and Accountability
The complexities involved with training and deploying GenAI systems result in a lack of transparency in the system's decision-making process. This lack of transparency can result in diminishing trust in the algorithm's capabilities, disputing public accountability, and ultimately hurting its further refinement.
A 2023 survey by TELUS International found that 71% of respondents would like more transparency about the use of GenAI systems.
Countermeasures to introduce transparency into the generative AI's inner workings, such as explainability features and proper documentation, can help develop a sense of accountability.
4. Social Implications
Ethical concerns surrounding the deployment of GenAI systems can arise due to their profound social implications, the misuse of which can cause economic disruptions, worsen existing social challenges, and impact employment opportunities.
According to the TELUS International survey, 57% of respondents were concerned about biases in GenAI impacting their professional and financial opportunities.
Addressing these dynamic social implications requires continuous monitoring of the social impacts generative AI brings about, and prolific collaboration with diverse policymakers serves as a way to deal with any unintended consequences.
Framework for Responsible Development
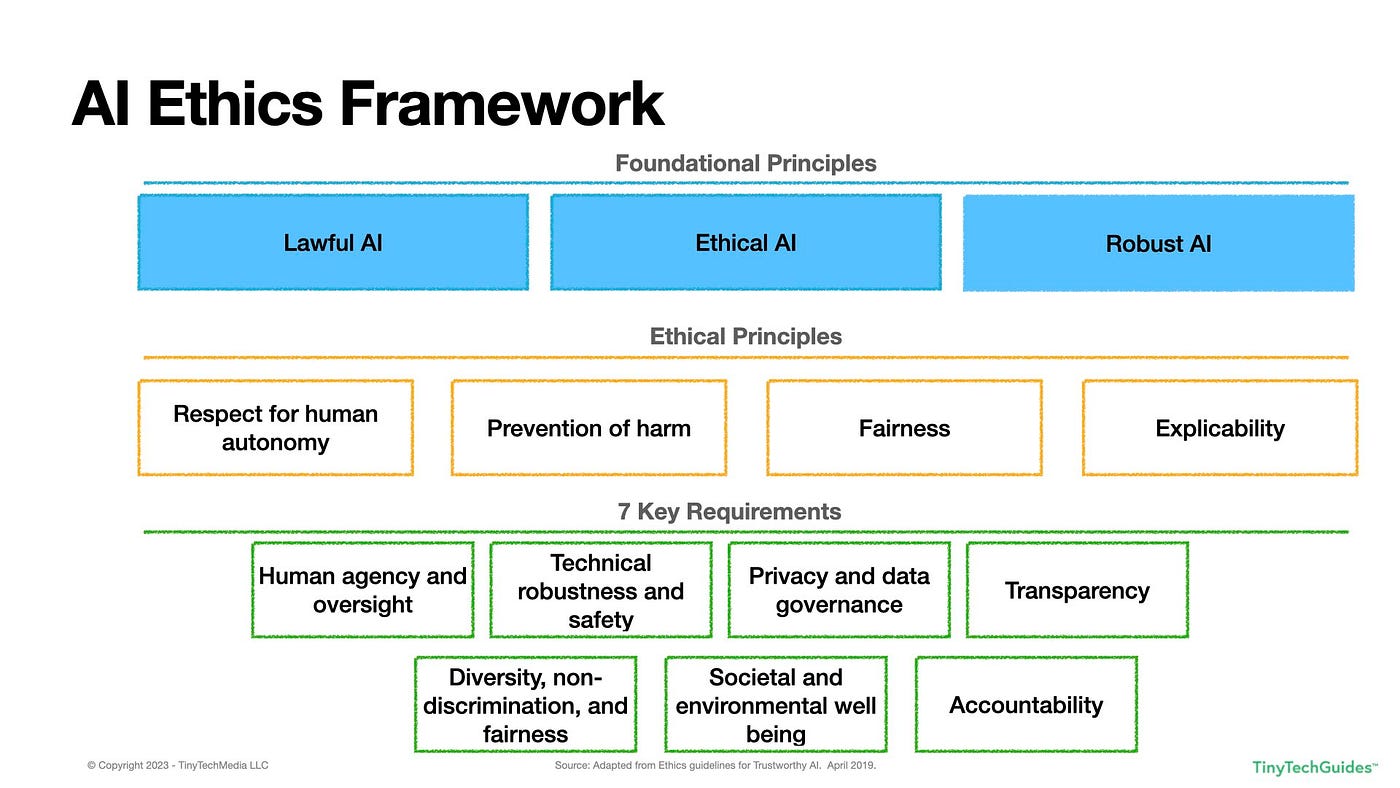
Constructing a robust framework is essential for responsible development. This framework should go over the strategies and safeguards needed to address the ongoing challenges associated with the widespread assimilation of GenAI systems. Let's discuss the most vital components:
1. Ethical Guidelines
The establishment of regulated ethical guidelines that prioritize transparency, privacy, and accountability will serve as a blueprint for GenAI developers, ensuring that ethical concerns are monitored and resolved at each stage of development and training.
2. Bias Mitigation Strategies
The development of bias mitigation strategies involves the adoption and monitoring of diverse datasets, helping to account for biases and reduce unfair outcomes. Techniques to mitigate biases based on real-world feedback can help ensure continuous improvement.
3. Transparency Measures
Transparency in the decision-making process of GenAI systems will help make outcomes more understandable to knowledge workers. Explainability features and proper process documentation will help develop accountability and the ability of users to understand how certain decisions are made.
4. User Privacy Protection
The protection of user privacy through dynamic encryption protocols and privacy regulations is non-negotiable in order to maintain the balance between the need for training data and the protection of sensitive information.
5. Ongoing Monitoring and Evaluation
Continuous monitoring and refinement of GenAI systems involves strict consideration of their impacts on the decisions made. Such monitoring and data governance evaluation protocols are necessary to identify and deal with any ethical concerns.
6. Community Engagement
Involving the community at large in the development process helps developers bring in diverse perspectives and identify potential ethical challenges, ensuring that GenAI systems are created with a more refined understanding of the societal challenges at hand.
7. Accountability Mechanisms
Establishing accountability mechanisms for addressing errors or unintended consequences includes the implementation of user feedback portals in order to implement corrective actions and make the decision-making process more transparent.
8. Education and Training
Providing education and training on the ethical considerations in GenAI systems is vital for creating a more informed and responsible community that actively participates in the ethical development and use of GenAI systems to keep developers accountable for their systems.
9. Adaptability to a Changing Landscape
A responsible framework involves regularly updating ethical guidelines and staying informed about evolving privacy standards to help mitigate developing ethical concerns.
10. Collaboration with Policymakers
Productive collaboration with policymakers helps establish strict regulations that can balance considerations of innovation with ethical challenges, ensuring that GenAI systems operate within ethical boundaries and promote responsible development.
Case Studies: AI-Powered Cyber Security

The widespread user base of Siemens necessitated the development of stringent and dynamic cybersecurity measures to account for the relentless anti-security measures being created daily, leaving no room for rest.
Through AWS Machine Learning, Siemens created a data lake that served as a secure method for the ethical development of forensic analysis, ensuring the proactive protection of sensitive user information while simultaneously allowing for the productive use of the data.
The platform was able to handle 60,000 potentially critical attacks per second, seamlessly safeguarding user data and facilitating responsible development.
Future Directions
The responsible development of GenAI systems is an ongoing process that requires continuous improvement. Future directions to address ethical concerns in GenAI include:
- Global Ethical Regulations: The establishment of global ethical standards for GenAI system development will ensure a consistent and responsible development approach around the world.
- Interdisciplinary Development: Interdisciplinary research bringing together expertise from fields such as ethics, law, and social sciences will help address the diverse and complex ethical challenges associated with GenAI system development.
- User-Focused Design: User-focused design principles will help ensure that GenAI systems are able to keep in line with user preferences and ethical expectations.
- Ethical Impact Evaluations: Evaluating the ethical impacts before deploying GenAI systems will help identify potential ethical concerns and mitigate them proactively.
Conclusion
Addressing the ethical concerns associated with GenAI systems involves the broad identification and resolution of the following challenges:
- Biases in GenAI algorithms
- Privacy issues due to GenAI's data-centric nature
- Lack of transparency
- The inability of laws to keep up with GenAI developments
By actively addressing ethical considerations at every stage of development and developing a robust framework for responsible development, GenAI systems can ensure that accountability is maintained throughout the process.
This is why AI-powered platforms like MarkovML establish responsible AI features to help knowledge workers develop AI systems and solutions that are transparent, accountable, and trustworthy.
Let’s Talk About What MarkovML
Can Do for Your Business
Boost your Data to AI journey with MarkovML today!