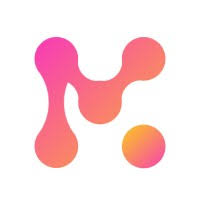
A Guide to Building Responsible GenAI Systems

As one of the key emerging technologies, GenAI systems are continuously attracting attention from organization leaders and decision-makers. In fact, according to a KPMG report, 74% of the business leaders surveyed admitted that GenAI would have a significant impact on their business over the coming years.
Although it is still considered a disruptive technology, it still has the potential to add immense value to business offerings in countless ways, according to 47% of business leaders.
Having said that, for GenAI systems to add any value to the business ecosystem, it is necessary to ascertain that it is built responsibly and within ethical and moral ambit.
Understanding GenAI Systems
GenAI, in simplest terms, can be considered as the next step in artificial intelligence. It can perform a variety of functions, like customer service, personalization of user experiences, etc., but it is primarily coveted for its analytical ability and power to converse in natural language similar to humans. It came from the need to process copious volumes of data with high precision, speed, and accuracy.
GenAI systems ingest a large volume of data and apply machine learning to it in order to produce holistic outputs that aren’t only technically correct but carry context and data subtleties as well. GenAI leverages the power of large language models to draw learning from and apply it to the queries raised in the system.
Key Principles of Responsible GenAI Development
Developing responsible GenAI systems requires careful attention to the way the model is trained, the datasets that are input as training features, and the established boundaries within which the AI system generates its responses. Covering the two principles listed below helps cover the ethical considerations of Generative AI:
1. Ethical Frameworks
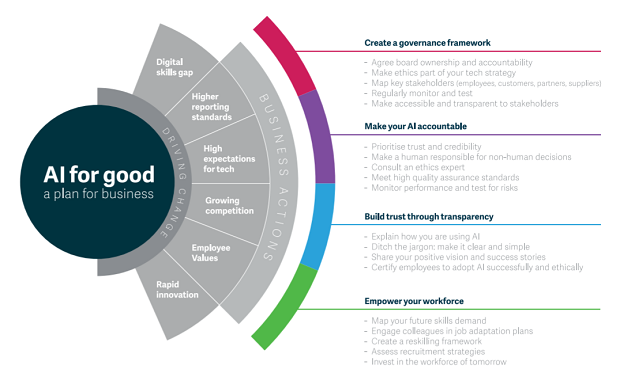
The ethical framework of developing responsible GenAI systems is the obligation of those who develop GenAI-based solutions. It needs to cover the following aspects:
- Caution and foresight during development that includes evaluation of potential drawbacks of deploying GenAI solutions through thorough risk assessments. Professionals need to follow the frameworks laid down by bodies like Nasscom’s Responsible AI Governance Framework, UNESCO’s Recommendation on the Ethics of AI, or OECD AI Principles.
- The disclosure of data and algorithm sources leveraged for technical and non-proprietary information utilized for modeling the GenAI solution.
- Taking accountability for the outputs generated by the Generative AI solution in forms that are accessible and intelligible.
2. Accountability and Oversight
Incorporating accountability and oversight in GenAI use is key to creating advanced solutions that are safe for use. In addition to considering the ethics of GenAI systems development, there is a need for professionals to imbue reliability and safety in systems. This can be done by strict adherence to privacy and user security regulations laid out by governing authorities.
Scheduled checks for data collection, processing, usage, and storage compliances need to be put in place for system audits. Additionally, accountability also involves promoting inclusion in Generative AI systems by ensuring that overfitting/underfitting does not occur, which could potentially introduce biases.
Ethical Considerations in Design and Deployment
Ethical considerations take precedence above all other considerations in developing GenAI systems. This involves training the models with the right datasets that naturally incorporate sensitivity, neutrality, and fairness into the model’s behavior.
The three major ethical considerations that enable GenAI fairness and consistency are listed below:
1. Mitigating Bias and Fairness
Existing datasets that are used to train large-scale GenAI models could potentially induce biases in the systems. This happens particularly in cases where the data scientists have limited access to large amounts of reliable data.
It is possible that the training models used for GenAI preparation already contain human biases in the form of repeated patterns or trends. For example, the hiring data at a firm may show an inclination for hiring a specific type of candidate more, not reflecting the industry-wide hiring practice for the specific role.
This pattern may get imported into GenAI systems as well if left unaddressed. As such, the screening results may omit the truly capable candidates solely because the patterns the GenAI learned from do not identify them.
2. Privacy-Preserving Design
It is essential to consider consumer privacy and data protection while developing generative AI systems. Right from the creation of new data to its disposal, all the privacy and data security laws and regulations should be built into the fabric of GenAI algorithms, enabling automated labeling and subsequent data treatment.
Since GenAI systems rely on user-generated data and inputs, they may be at risk of processing information that is private, sensitive, or even confidential (it may happen because of users’ mistakes).
By taking the aid of security frameworks and data handling mandates laid out by the governing authorities, it is possible to ensure that sensitive user data remains secure throughout its lifecycle in the GenAI systems.
3. Security Measures
For the question of security, let’s refer to a few numbers revealed by a Zscaler report:
- 95% of the organizations already use some type of GenAI solutions.
- 57% of the organizations allow GenAI use without any major restrictions.
- 89% of the organizations also agreed that GenAI carries a potential risk to their organizational security.
The discrepancy in numbers spells out a dire concern: there is a wide gap between GenAI adoption and its inbuilt security measures.

Problems like data leakage and system breaches further underscore the importance of introducing robust security wireframes at the heart of Generative AI.
Human-Centered Design and Collaboration
In a nutshell, human-centered AI design refers to the GenAI systems design approach that puts the needs and requirements of its users at the front and center of the entire process. The concept of HCD (human-centred design) in AI stems from the need for creating intelligent systems that augment human work rather than displace it.
The resulting systems aim to preserve human control over the process in a way that is transparent, equitable, and respectful of the privacy of the end-users.
1. User Involvement
User involvement is key to developing GenAI solutions that are considerate and performant with respect to goals and user needs. Stakeholders from the leadership teams, IT teams, end users and technology partners can come together to collaborate towards developing a responsible GenAI solution.
It is also a highly effective way to enhance the end-user experience and satisfaction from the use of the final product. Consequently, user involvement also helps create low-code environments where the stakeholders – whether technically adept or not – can contribute their inputs to the whole. An offering of different perspectives brings completeness to the result.
2. Interdisciplinary Collaboration
GenAI systems draw from large language models or other high-volume data for deriving training and insight. The application of these solutions to the societal fabric must follow an interdisciplinary approach that weighs biases, technical inaccuracies, and the impact of errors on concerned policies.
The deployment needs to be overseen by a collection of authorities, stakeholders, and representatives of the society that help vet the ethical dimensions of the GenAI solution. Model autonomy needs to be thoroughly examined for problematic outcomes stemming from imbuing biases through generalized data ingestion.
The incorporation of moral and social values into the very fabric of the GenAI solution thus becomes important for eliminating potential issues like privacy compromises, offensive results, etc.
Best Practices in Responsible GenAI Development
The ever-expanding capabilities of GenAI solutions are a boon for the world of business. However, the scope of these solutions must be governed by firewalls that fiercely protect the users against potential risks, such as data breaches. Use the best practices below for developing responsible GenAI systems:
1. Explainability and Interpretability
Incorporating explainability is key to understanding the decision-making process of a GenAI system. In situations where it delivers controversial or problematic results, it becomes crucial to identify the datasets, processes, and methods that led to the conclusion.
The high interpretability of GenAI systems is a crucial requirement to understand the data correlations, patterns, and trends that the AI system has identified and is using to generate results. It helps with streamlining the model to remove system biases and inaccuracies.
2. Regular Audits and Assessments
To ensure that the new data that a GenAI system ingests is compliant with ethical standards and privacy policies, audits and assessments are necessary. It is best to create schedules for conducting model audits that generate reports on the degree of deviation that a GenAI system has experienced by training from recent data.
Assessments are a key process to understand a variety of performance aspects of a GenAI system, like accuracy, data security, deployment speed, and more. Period assessments help professionals ensure that the responsible GenAI systems are performing to the benchmarks and standards prescribed.
3. Transparency in Model Training
The transparency of a GenAI model allows users to peer inside it and see its internal workings. An opaque model reveals no insights regarding the analysis methods and processes used. On the other hand, a transparent model is easy to understand, interpret, tweak, and finetune according to organizational requirements.
Issues such as complexity, difficulty in creating explainable solutions, and risk concerns push an AI model to become a “black box” that allows little visibility into its function. Consider leveraging the power of data visualization tools to enhance transparency and visibility into a model, enhancing their accessibility as well.
Continuous Learning and Adaptation
The factor that makes generative AI systems so competent is their ability to continuously learn and adapt to the new information they ingest. A good example is a comparison between static AI models that learn from a fixed dataset. It stunts the capability of the model to understand evolutionary trends concerning a subject, resulting in outdated outputs that may not remain relevant.
On the contrary, GenAI continues to learn from each interaction it holds with each user. The input data enables it to update its resources and knowledge bank, refine its algorithms, and adapt to the change more readily. The dynamic approach of GenAI systems helps preserve creativity and innovation that almost mirrors human capabilities to learn from each experience.
With that said, continuous learning isn’t without its own set of drawbacks that must be addressed before a GenAI system can be deemed usable. One such phenomenon is catastrophic forgetting, which causes the systems to “forget” the original knowledge when a new one appears.
Additionally, the scalability of GenAI becomes a real-world issue when the continuously accumulating data poses storage and management concerns for the enterprise.
In the midst of all the boons and banes, GenAI still remains the most sought-after technology that enterprises require for high-precision analytics.
Future of GenAI Systems
GenAI is an emergent technology that has been labeled disruptive. However, the full scope of transformational benefits that generative AI has to offer is yet to be realized.
According to the Salesforce State of IT Report 2023, it was revealed that 9 out of 10 CIOs already admitted that GenAI has gone mainstream. As the role of automation and AI increases in the business realm, GenAI will continue to grow and expand in its capabilities.
With that said, it is still a challenge to leverage GenAI for tasks such as anomaly detection in factories, for which the standard AI models are better equipped to perform. The future of GenAI systems lies in a diverse set of industries, such as healthcare and education, where a massive amount of research and data condensation is required at scale.
Generative AI has the potential to process large amounts of information, which is the cornerstone for streamlining workflows in data-intensive industries such as customer service.
The constant evolution of GenAI systems requires an equal investment in AI ethics and responsible development. A concrete policy that outlines the moral and ethical considerations of developing generative AI should be created.
Conclusion
In the end, artificial intelligence learns from the data that is input into it. The quality of its results depends on the quality of its inputs. For developing responsible generative AI, it is first necessary to outline the definitions of neutral data and bias-free datasets that would be used to train the GenAI systems.
The ultimate onus of responsibility and accountability in GenAI lies upon the entire stakeholder chain. It is required to put policies, assessments, audits, and protocols in place that flag undesirable AI model behavior and alert the developers to retrain the systems.
Additionally, data scientists are also required to thoroughly assess dataset quality and neutrality before queueing it up for GenAI systems to ingest. It is possible to leverage AI-based mechanisms to detect and flag model behavior problems.
On that front, MarkovML provides you with a robust GenAI wireframe, on top of which you can build reliable solutions for your enterprise. Give your organization the capability to organize ML data with intelligent data cataloging features. Leverage this data for developing GenAI apps using the no-code app builder with enhanced governance protocols.
MarkovML also provides data privacy and security features to eliminate data-related risks. To understand MarkovML’s offerings in deeper detail, visit the website.
Let’s Talk About What MarkovML
Can Do for Your Business
Boost your Data to AI journey with MarkovML today!