Roles and Responsibilities of a Data Scientist in 2024

The role of a data scientist has emerged as a cornerstone in unlocking insights from massive volumes of data. Glassdoor consistently ranks this specialized position among the top 50 jobs in the US.
Data science stands at the intersection of statistics, technology, and business acumen. A data scientist is a professional with the skills to collect, analyze, and interpret large, complex datasets. They extract actionable insights from raw data, transforming it into a strategic asset for business decision-making.
The surge in demand for data scientists is striking, with a projected growth rate of 35 percent from 2022 to 2032, outpacing the average for all occupations significantly.
For Gen Z, entering the job market with a fervor for innovation and technology, data science jobs have become a top career choice.
This blog post aims to demystify the roles and responsibilities of data scientists, their challenges, and future trends.
Key Skills and Qualifications of a Data Scientist
Becoming a proficient data scientist is as much about acquiring a diverse skill set as it is about understanding data science. Central to this journey are a few key skills and qualifications that define the effectiveness and success of professionals in this field.

1. Technical Skills
Technical expertise forms the foundation of a data scientist's skill set. Fundamental proficiency in programming languages such as Python or R is key for data manipulation and analysis. Equally important is a strong grasp of SQL for effective database management and retrieval.
Furthermore, a profound understanding of machine learning techniques and algorithms is imperative, enabling the development of predictive models based on existing data.
A data scientist should also be familiar with big data technologies like Apache Hadoop or Spark, crucial for handling extensive data sets.
Additionally, the ability to utilize data visualization tools like Tableau or Power BI is essential for presenting complex data in an easily understandable format, facilitating effective communication with stakeholders.
Proficiency in statistical analysis and experimental design is necessary to draw meaningful insights and ensure reliable results.
In the current landscape of unstructured data, an understanding of natural language processing (NLP) and text analytics is increasingly valuable, allowing data scientists to extract insights from text-based data sources.
Finally, knowledge of cloud computing platforms like AWS or Azure is becoming essential, given the increasing migration of data storage and processing to the cloud. Data scientists must be adept at working within these environments.
2. Mathematical and Statistical Skills
Data science is deeply rooted in mathematics and statistics. A strong grasp of statistical tests, distributions, and probability theory is essential for interpreting data and deriving meaningful conclusions.
Moreover, understanding various machine learning concepts necessitates a solid foundation in linear algebra, calculus, and other advanced mathematical disciplines.
3. Domain Knowledge
While technical prowess is key, the ability to apply this expertise to specific industries or domains can set a data scientist apart. Domain knowledge not only helps in understanding the nuances of the data specific to that field but also in communicating findings that are actionable and relevant.
Possessing contextual understanding is invaluable, whether it's finance, healthcare, retail, or any other sector.
4. Communication and Collaboration Skills
The ability to effectively communicate complex data insights to stakeholders is critical. This includes proficiency in data visualization tools and the capacity to translate technical jargon into clear, concise, and actionable business language.
Collaboration skills are equally important, as data scientists often work in interdisciplinary teams, requiring them to be adept at navigating group dynamics and integrating inputs from various sources.
Roles and Responsibilities of a Data Scientist
The role of a data scientist is multifaceted, encompassing a range of responsibilities crucial in transforming raw data into actionable insights. Each responsibility, from data collection to model deployment, is a step in making data meaningful.

1. Data Collection and Cleaning
Data scientists gather data from various sources, including internal databases, online repositories, or external datasets. However, raw data is often unstructured and replete with inaccuracies or irrelevant information.
Consider a data scientist working in healthcare. They may collect patient data from electronic health records, but this data could be riddled with inconsistencies, missing values, or irrelevant details. The scientist's task is to clean and preprocess this data, ensuring it's accurate, complete, and formatted for analysis.
2. Data Analysis and Visualization
Once the data is prepared, it must be analyzed to uncover trends, patterns, and correlations. Data scientists use statistical techniques and tools to conduct this analysis. Visualizing the data is also key to making the insights accessible and understandable.
For instance, a data scientist might analyze customer purchase data in a retail company to identify buying trends. They might use visualization tools to present these trends in an easily digestible format, helping the marketing team to strategize effectively.
3. Model Development
Developing predictive models is a core task. This involves selecting appropriate algorithms and techniques to create models that predict future trends or behaviors based on historical data.
For example, in a financial institution, a data scientist might develop a model to predict credit risk based on a customer's transaction history, demographic data, and other variables.
4. Model Deployment and Monitoring
Deploying the model into a production environment is where data science provides tangible value. Once deployed, these models need continuous monitoring and maintenance to remain accurate and effective.
For example, in e-commerce, a data scientist might deploy a recommendation system model to suggest products to users. They must constantly monitor and tweak the model for optimal performance, ensuring it adapts to changing customer preferences and inventory.
5. Continuous Learning and Improvement
The field of data science is ever-evolving. Therefore, continuous learning is key to staying abreast of new technologies, algorithms, and industry best practices.
For instance, a data scientist in autonomous vehicles must continually learn about new advancements in machine learning and sensor technology to enhance the models used in self-driving cars.
Challenges Faced by Data Scientists
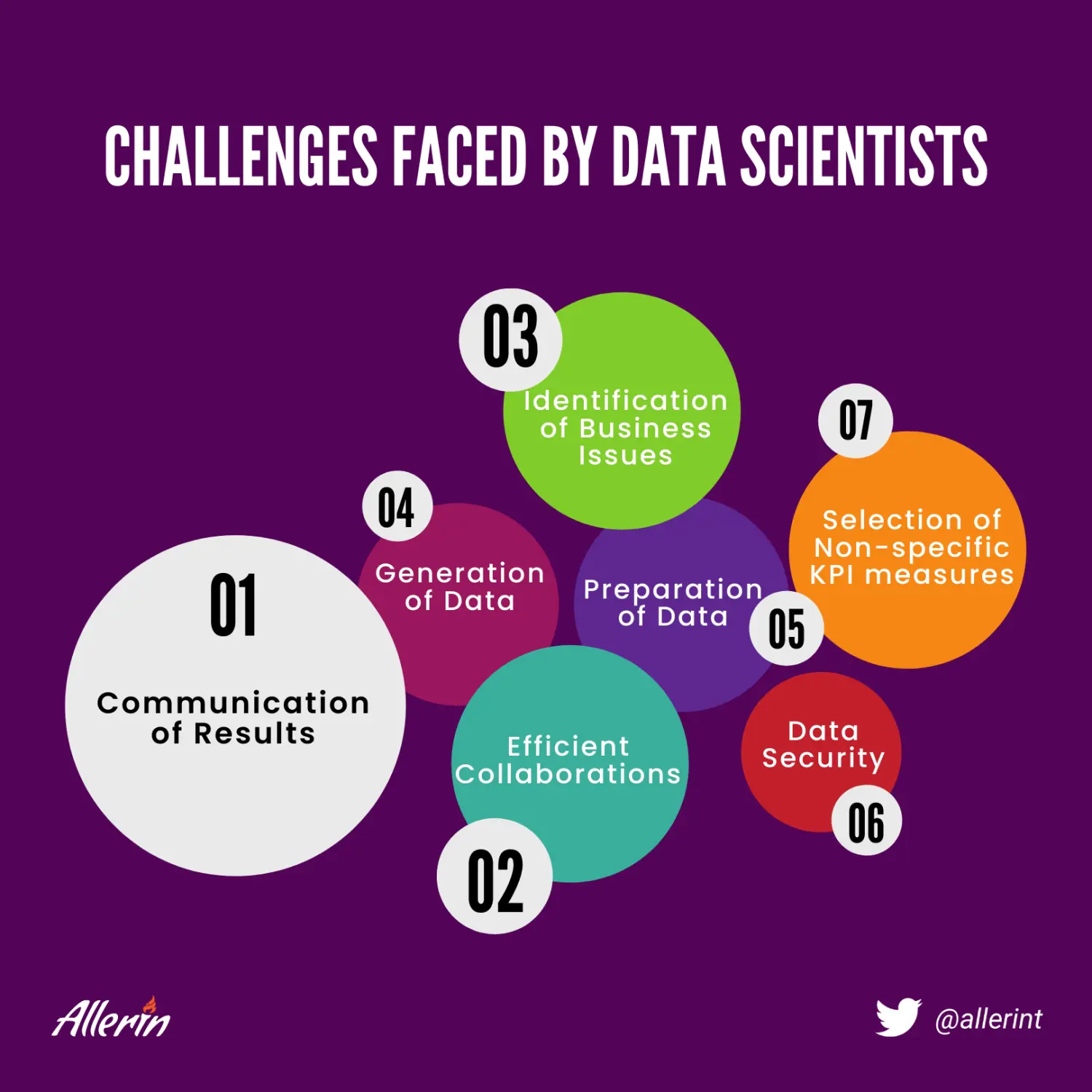
Data Scientists, pivotal in transforming data into strategic insights, face challenges that test their skills and adaptability. These challenges range from technical hurdles to organizational dynamics:
1. Data Quality Assurance
Ensuring data integrity is paramount. Data scientists often grapple with datasets plagued by errors, inconsistencies, or incompleteness. Cleaning and validating such data to make it usable is both time-consuming and complex.
2. Navigating Big Data Analytics
The sheer volume of data demands proficiency in advanced analytics. Techniques like distributed computing and parallel processing are essential but require specialized knowledge and tools, posing a steep learning curve.
3. Upholding Data Security
With increasing data breaches, ensuring the security and privacy of data is crucial. Data scientists must implement robust security measures to protect sensitive information from unauthorized access or theft.
4. Effective Communication with Stakeholders
Translating complex data insights into understandable, actionable information for audiences is a recurring challenge. It requires a unique skill set to articulate technical findings clearly and concisely.
Future Trends in Data Science
As we advance into 2023 and beyond, the field of data science is poised to undergo transformative changes.

Here are some of the key future trends that data scientists and industry observers should be attuned to:
1. Increased Use of AI and Machine Learning
The application of AI and machine learning in data science is set to expand. This trend will empower data scientists to handle larger datasets and enhance the accuracy of their predictive models.
2. Democratization of Data Science
Data science is becoming more accessible, extending beyond traditional domains. This shift opens the field to a diverse range of professionals, enriching the discipline with varied perspectives and approaches.
3. Rise of Small Data and TinyML
A growing focus on small data and TinyML marks a shift from the dominance of big data. This involves analyzing more compact datasets and leveraging machine learning in low-power devices, broadening the scope and application of data science.
4. Advancements in AutoML
Automated Machine Learning (AutoML) is streamlining the process of developing machine learning models. This trend is crucial for enabling those without deep technical expertise to utilize machine learning tools, significantly broadening the user base.
5. Heightened Focus on Data Privacy and Security
As data generation increases, so does the importance of data privacy and security. Data scientists are increasingly expected to prioritize protecting sensitive data, adhering to stringent privacy standards and security protocols.
The Importance of Data Scientists and Their Impact on Business
Data Scientists are essential catalysts in the contemporary business landscape, impacting how organizations operate and thrive. Using their expertise in predictive analytics and machine learning, data scientists refine business strategies and equip organizations with a competitive advantage in a data-centric market.
Amidst this, Markov emerges as an invaluable asset for data scientists. It simplifies the journey from raw data to actionable intelligence, offering deep data insights and automated workflows without necessitating coding skills.
MarkovML empowers data scientists with tools for effective data analysis, artifact management, no-code GenAI app creation, and responsible AI evaluators. It enhances their work's efficiency and broadens their capabilities' scope.
Let’s Talk About What MarkovML
Can Do for Your Business
Boost your Data to AI journey with MarkovML today!