Social Sentiment Analysis Using Sentiment Compound Valence
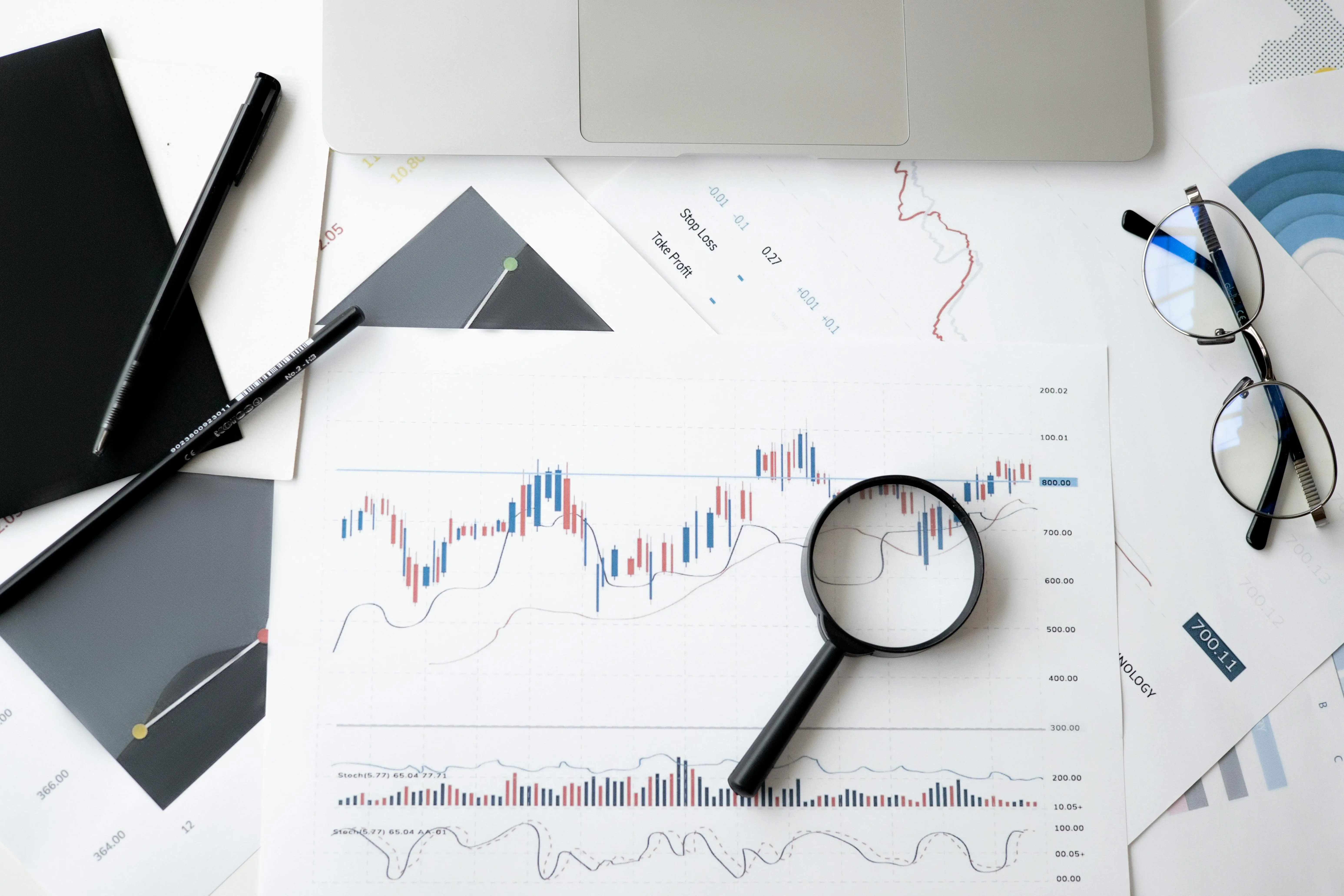
Did you know that a majority of the purchase decisions a customer makes are driven by the subconscious and sentiment? Sentiment analysis is crucial for businesses to understand how to approach their customers to make a sale.
Social sentiment analysis thus pertains to the study of consumer sentiment as it appears on user-generated content on social media channels. To do so, businesses use a metric called “compound valence”, which measures the degree of sentiment in a sentence.
Let’s understand how sentiment compound valence can make a difference in social sentiment analysis.
What is Sentiment Compound Valence?
Sentiment compound valence is a key metric used in social sentiment analysis that represents the aggregated sentiment of a piece of text. Negative sentiment is marked as (-1), and positive as (+1), signifying 'extremely negative' and 'extremely positive' respectively.
Compound valence has four major components:
- Positive score: The portion of phrases that convey positive sentiment.
- Negative score: The portion of phrases that convey negative sentiment.
- Neutral score: The portion of phrases that convey no strong sentiment.
- Compound score: A combination of positive, negative, and neutral scores.
Although sentiment compound valence is not conceptually different from traditional sentiment analysis, there is one major difference. While the traditional method provides a contrasting positive or negative result on sentiment, the compound valence score provides aggregated feedback that highlights the overall emotional score considering all positives, negatives, and neutrals.
Why Does Sentiment Compound Valence Matter?
Several factors make sentiment compound valence pivotal for business decisions:
1. Nuanced Understanding of Sentiment
Compound valence score is an aggregate of all the negatives, positives, and neutrals in user-generated text. It helps glean a more nuanced understanding of the expression that represents the overall sentiment of the customer. It is more holistically representative than basing the result on the predominant sentiment in a sentence.
2. Improved Accuracy in Sentiment Analysis
Compound valence score measures the degree of positivity or negativity of each word in a user-generated sentence. The final score depends on the aggregate of all these positives and negatives and is expressed numerically. This allows for a more granular sentiment analysis of UGC rather than just ascribing a vague “positive”, “negative”, or “neutral” label to a sentence.
3. Consideration of Context in Sentiment Evaluation
Compound valence calculations also consider aspects like grammatical structure, linguistic nuances, and word order to determine the context of a piece of text. This process augments the granular analysis of sentiment by providing a background for the emotions to be scored appropriately as positive, neutral, or negative.
4. Enhanced Decision-Making for Businesses
The ultimate goal of sentiment analysis is to be able to make the right business decisions. Compound valence is a numerical score that highlights how negative or positive the overall customer sentiment is, making it easier to prioritize actions. Traditional sentiment analysis only deals with labels that aren’t as insightful.
How AI is Revolutionizing Sentiment Analysis
AI provides businesses with sentiment analysis tools and techniques that are faster, more accurate, and scalable for processing large volumes of data.
There are three key ways in which AI is transforming sentiment analysis processes:
1. Role of Advanced NLP Models
With the use of pre-trained language models (like BERT), it is possible to enhance the accuracy and performance of tasks. These models are trained on large-scale texts which helps them extract the context-specific meaning in spoken language.
When these models are adjusted specifically for sentiment analysis, they can connect the words in a sentence and accurately detect sentiment, negations, modifiers, and other language cues that basic analyses might miss.
2. Integration of Machine Learning Algorithms
Machine learning algorithms are designed to self-learn, applying the information gleaned from labeled data to categorize and classify unlabeled data. Integrating machine learning algorithms into sentiment analysis can help develop customized models by training them on domain-specific data.
This enables the ML models to identify industry-specific language, terminology, and sentiment expressions which helps with a more accurate and actionable sentiment analysis.
3. Real-time Insights and Adaptive Learning
Real-time sentiment analysis is crucial for high-stakes industries (such as healthcare or finance) where customer opinion can quickly make or break a brand image. Leveraging AI-based adaptive learning models can help with processing real-time social media data like tweets, posts, and stories, helping businesses quickly extract overall sentiment and respond to consumers promptly.
Adaptive learning can help the AI models adapt to changing language patterns and word usage, making them more accurate and relevant to trends for social sentiment analysis.
Practical Use Cases
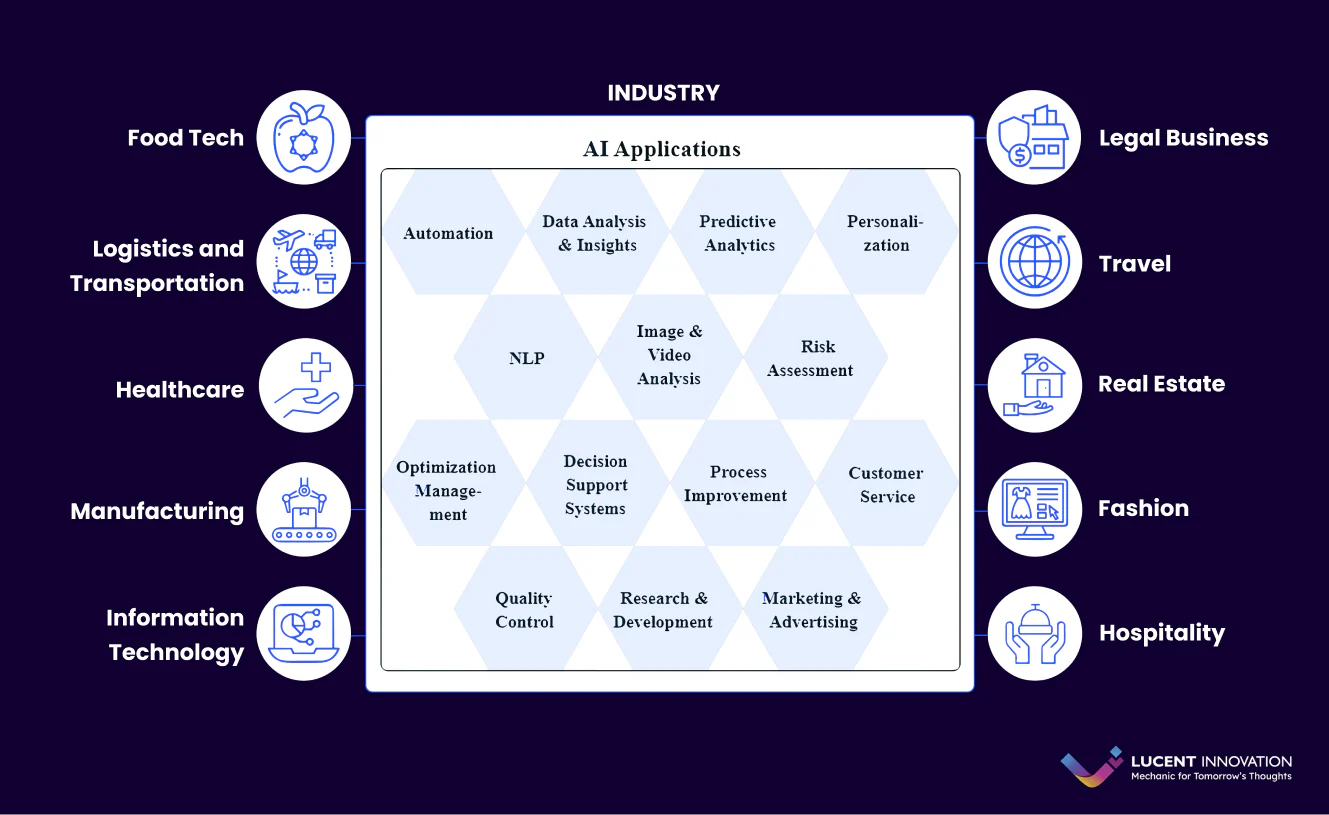
Social sentiment analysis makes itself significant across several crucial use cases:
1. Brand Reputation Management
Establishing a sound brand reputation is extremely important to help boost sales and enhance customer relationships in the long run. Sentiment analysis makes it possible for a brand to manage its reputation online, where so much could go wrong with one ignored negative feedback.
Sentiment analysis can help brands manage their reputation by:
- Identifying positive and negative sentiments to help mobilize customer service promptly whenever needed.
- Monitoring sentiment across various social channels like Twitter, Instagram, Facebook, etc. to gain insight into the difference in brand perception across demographics.
2. Product Development Insights
Sentiment analysis is the cornerstone of gleaning product feedback from social media. It can help brands with:
- Prioritizing certain features in products that customers like or dislike, based on how they are expressing feedback on social media. Using this insight, it is possible to prioritize favored features and remove unwanted ones.
- Analyzing user sentiment towards competitor products and comparing it with your own. It helps establish a baseline for customer expectations and helps with product development for better responses.
- Assessing market demands. It helps track the talk of emerging trends and technologies, helping brands identify opportunities for innovation.
3. Social Media Marketing Strategies
It is possible to improve your social media marketing campaigns with sentiment analysis in the following ways:
- Optimize your content strategies by analyzing sentiment on your social posts and advertisements. It is a great way of identifying which visuals or messaging are attracting the most customers.
- You can monitor a campaign's performance using sentiment analysis in real time if you have AI-based tools. You can identify, for example, which promotional hashtag receives exciting responses, and which ones receive backlash from the consumers.
Future Implications
Innovation never stops, and the field of sentiment analysis is also undergoing constant improvement and evolution. Several future trends in sentiment analysis and compound valence point towards a more accurate understanding of consumer opinion about a brand and its products:
Aspect Based Sentiment Analysis (ABSA)
ABSA is a technique to identify consumer sentiment in a more granular way by identifying specific aspects or attributes in the text that determine the associated emotion. This way, brands can identify the subtle nuances of expression, enabling better finetuning of offerings, products, and services.
Multimodal Sentiment Analysis and Compound Valence
While traditional sentiment analysis works majorly with a single-mode input (such as text or image), multimodal analysis can generate a combined sentiment analysis by studying inputs across various modes like text, audio, images, videos, etc.
This way, compound valence scores can more holistically capture public sentiment for products and brands.
Conclusion
Sentiment analysis is a necessary business operation that helps brands identify what approaches are helping them create better relationships with their target audience. Leveraging sentiment compound valence helps brands numerically understand the sentiment of their consumers on a scale, which enables better business decisions.
Compound valence scores can be augmented using AI-based tools such as NLP and machine learning to generate industry-specific results, giving even deeper insight into consumer sentiment. The best part is that these AI sentiment analysis tools can be customized using simple AI-based platforms like MarkovML.
MarkovML provides highly efficient data intelligence and management features such as no-code auto-EDA, collaborative reporting, and intelligent data catalogs. Using these features, your business can easily unlock deep insights from consumer sentiment, eliminate data siloes and establish streamlined and organized data structures within your systems.
Let’s get on a call to explore how our solutions can help your business understand its target audience better.
Let’s Talk About What MarkovML
Can Do for Your Business
Boost your Data to AI journey with MarkovML today!