Exceeding Efficiency: Automation of Machine Learning Workflows
In the evergreen field of Machine Learning, Automation Workflow as a concept has taken root, helping to reshape the landscape of model development. According to a McKinsey & Company report, 31% of businesses have at least one function that is fully automated.
Automation Workflow in Machine Learning refers to the systematic process of automating various stages of the ML roadmap, increasing efficiency in how input data is processed and models are trained.
This article goes into the intricacies of Automation Workflow concepts in ML, exploring its various components, advantages, and the tools available for utilization.
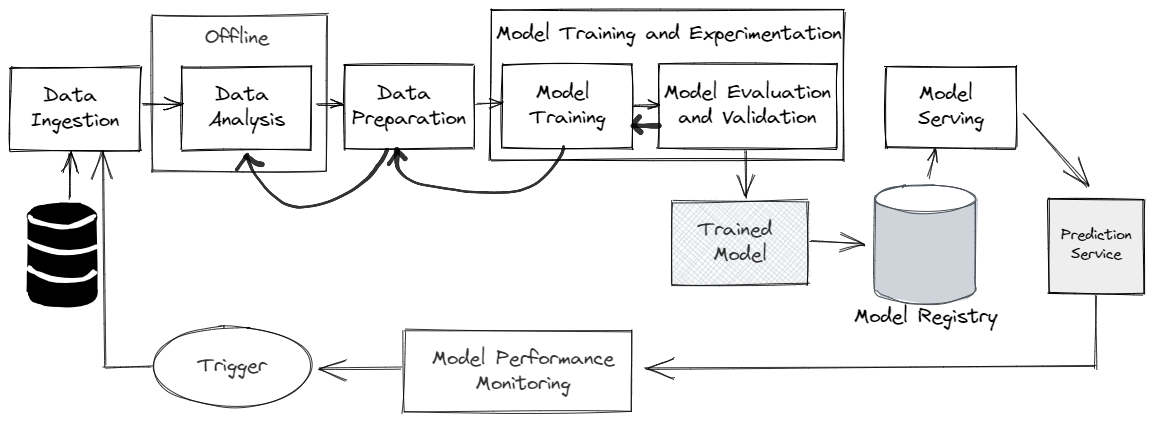
The Concept of Automation Workflow in Machine Learning
The concept of Automation Workflow in Machine Learning is a shift in how models are created and implemented. This streamlining makes development faster and enhances efficiency in the ML process.
Automation Workflows ensure a more standardized approach, helping to reduce the burden on data scientists and knowledge workers.
By automating repetitive yet crucial tasks, organizations can allocate resources to tasks that require more human thought and insight. According to a Deloitte survey, organizations already implementing or scaling automation report a cost reduction of 24%.
Key Components of Automated Machine Learning
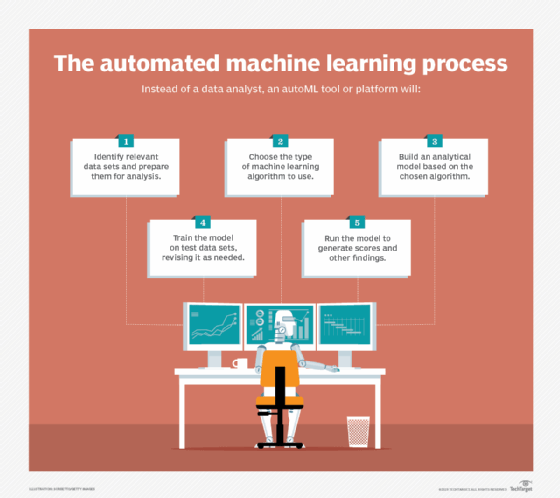
- Data Preprocessing and Cleaning: Automation Workflows address the time-consuming tasks of preparing and cleaning data, helping ensure that it meets the prerequisites for competent model training. This phase typically involves accounting for missing values, handling outliers, and organizing data to be ready for analysis.
- Feature Engineering: The efficacy of ML models often depends on the quality of their data features. Automation Workflows streamline and automate the creation of relevant features, optimizing model inputs for better model performance.
- Model Selection and Hyperparameter Tuning: Selecting the appropriate model and adjusting hyperparameters are vital for optimized performance. Automation Workflows automate this process by experimenting with various combinations in order to identify the best configurations.
- Model Deployment and Monitoring: Automation Workflow Tools ensure a consistent and smooth shift toward the production phase. Once deployed, they continue to monitor the model's performance and make adjustments to ensure efficiency.
Benefits of Automated Machine Learning Workflows
The incorporation of Automation Workflow frameworks has a lot of benefits. Apart from saving time in model development, Automation Workflows reduce the risk of human errors.
A 2021 Deloitte analysis also reports that, due to automation, respondents anticipated an average cost reduction of 24% over the next three years.
Time saved through automation can be reallocated to tasks requiring more forethought, such as exploring advanced modeling algorithms or solving complex business problems. This helps increase productivity and gives organizations the agency to respond to any changing business needs.
Tools and Frameworks for Automated Machine Learning
- AutoML in Cloud Platforms: Cloud platforms offer AutoML services to automate various ML tasks to make them more accessible to users with differing levels of knowledge and expertise. According to a Grand View Research report, enterprise companies are now shifting to cloud-based infrastructures.
- Open-Source AutoML Libraries: Open-source libraries such as PyCaret and TPOT provide flexible environments for implementing Automation Workflow concepts in ML. These libraries empower data scientists and knowledge makers with the ability to adjust their workflows to specific project requirements.
- Commercial AutoML Platforms: For enterprises with specific needs, commercial platforms provide advanced automation features and support. Platforms such as MarkovML are designed to cater to different requirements, providing a comprehensive suite of Automation Workflow tools.
How do you choose the Automated ML Tool?
According to a report from Deloitte, only 37% of organizations report that they have appropriate standards overseen by a capable IT team in charge of automation. That being said, selecting the right Automation Workflow tool is a major decision influenced by various factors:
- Use Case and Requirements: The tool's capabilities should be congruent with the specific requirements and goals of your ML project. Different use cases demand different functionalities, which will, in turn, require proper selection.
- Ease of Use: The tool should be user-friendly and in line with the skill level of your team. An intuitive user experience can significantly impact the rate of adoption of the tool within an organization.
- Supported Algorithms: It is important to ensure that the tool supports a diverse set of algorithms to address the complexity of a variety of ML problems. With a larger range of algorithmic support, the tool can adapt to more diverse use cases.
- Customization and Control: The ability to adjust the workflow to specific project needs enhances adaptability, which is why considering the level of customization available in a tool is imperative for its easy adoption.
- Scalability: The scalability of the tool plays a big role in easy integration if your project involves large datasets or requires extensive computing resources. A scalable tool ensures that it can handle growing resources as the project expands over time.
- Integration with Existing Infrastructure: Smooth integration with your current infrastructure is essential to avoid any major disruptions. By evaluating how the tool integrates with your systems, you can determine if it is feasible as an adaptable operation.
- Deployment and Model Serving: Evaluate the tool's capabilities for entering models into production and support for model serving. Efficient deployment mechanisms and robust model serving are necessary for future real-world applications.
- Interpretability and Explainability: Model interpretability is crucial for understanding predictions and reverse-engineering decision paths. Choosing a tool that provides transparent insights into model decisions helps build trust and reliability.
- Cost and Licensing: Understanding the cost structure and licensing terms associated with the tool helps align the addition within your budget. By considering long-term costs, you can make use of tools that have the ability to adapt and scale as your project grows.
- Community and Support: Consider the presence of the tool's community and the availability of support resources for it. An active community and strong product support will be able to provide valuable insights and ensure timely resolutions for any issues.
- Security and Compliance: Ensure that the tool is in line with security standards and compliance regulations that are enforced in your industry. When dealing with sensitive data, security is of the utmost importance.
- Trial and Evaluation: Many tools offer trial periods, which are immensely helpful in assessing how well the tool works within your project boundaries. A trial allows hands-on exploration without the need for any long-term commitments or spending.
Real-World Applications

The advent of Automation workflow in ML has led to widespread applications across various industries, showcasing its versatility and impact. According to a McKinsey & Company report, automation has been heavily adopted by 88% of financial services and 76% of the technology sectors.
A Statista report stated that 81 percent of clinicians surveyed in China agreed that the volume of patient data available to them is overwhelming. To counter this problem in healthcare, Automation Workflow tools can aid in diagnosing diseases and enhance their speed and accuracy.
These real-world applications highlight the effectiveness of Automation workflow in solving complex issues in a variety of fields.
The Future of Machine Learning Automation
The Automation Workflow concept in ML now functions as a reliable way to speed up development stages and enhance the reliability of ML models. By carefully selecting the right Automated ML tool based on use cases, requirements, and other critical factors, businesses can unlock the true potential of Automation Workflows.
Innovative AI-driven platforms like MarkovML help save time and resources by automating mundane data tasks, building workflows outside of working hours, and reducing the risk of human errors. The ability to automate even the most complex data workflows facilitates seamless collaboration for data-centric decision-making.
Let’s Talk About What MarkovML
Can Do for Your Business
Boost your Data to AI journey with MarkovML today!