
MarkovML Propelled Spectrum Labs Achieve Content Moderation At Scale
Spectrum Labs is a leading SaaS provider offering an innovative content moderation solution for platforms across domains and languages. Their product uses state-of-the-art machine learning technology to identify toxic content, encompassing diverse categories of harmful behaviour such as sexual abuse and hate speech.
Spectrum Labs' Content Moderation Challenge
Spectrum Labs provides a platform that enables businesses to uphold a safe and inclusive online environment. By effectively detecting and mitigating toxic content, the platform ensures a positive user experience while also safeguarding brand reputation.
However, it is challenging as certain phrases that might be considered harmless flirting banter in a dating app can be flagged as harmful when used in children's apps.
With a comprehensive suite of behavioral models, Spectrum Labs delivers cutting-edge content moderation capabilities, making it a valuable ally in promoting digital well-being and fostering responsible online communities.
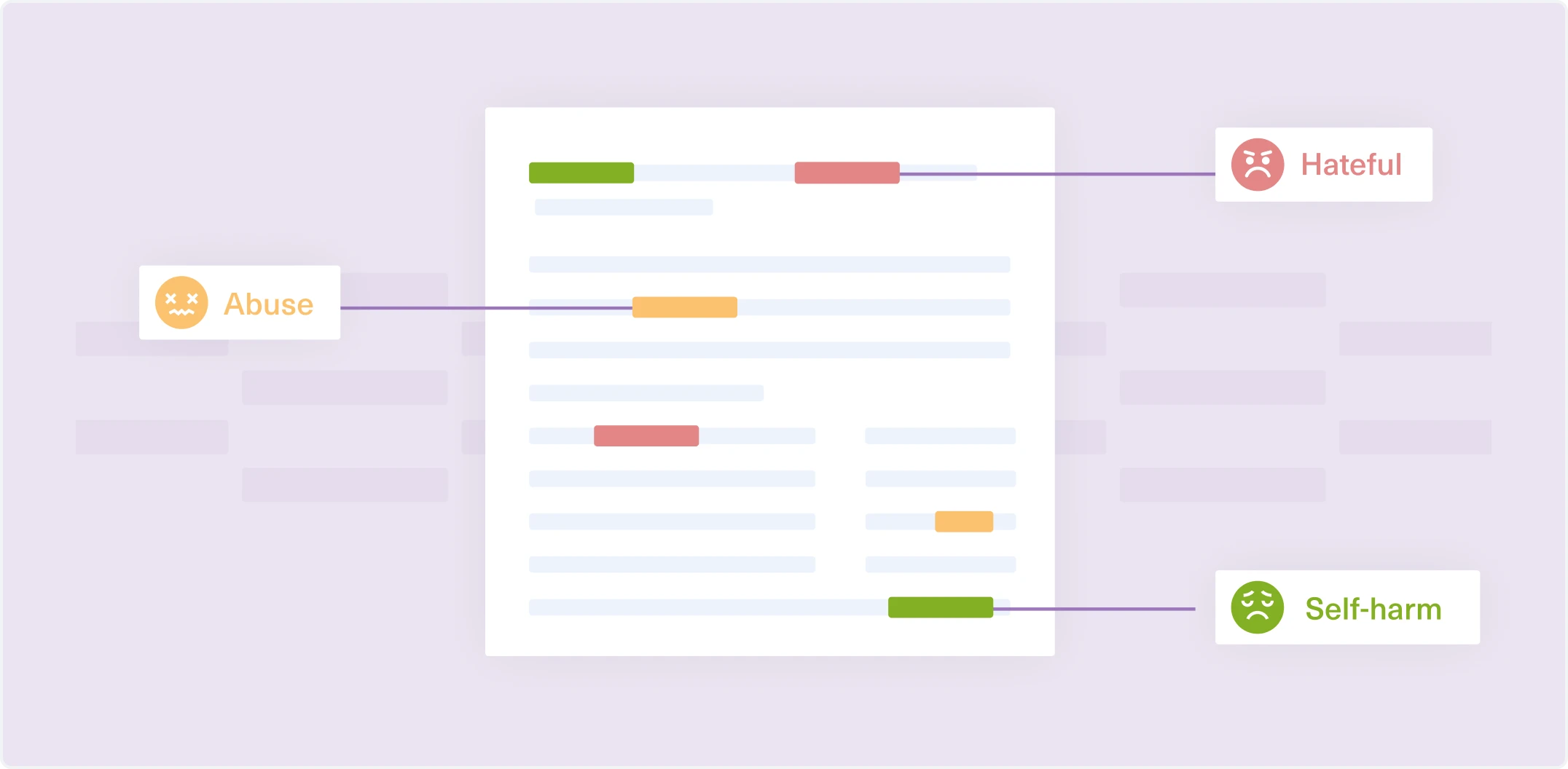
Key Difficulties
- Centralized Data Management: Streamline data management by centralizing datasets, models, metrics, and evaluations, ensuring easy discovery, effortless collaboration, and consistent compliance with lineage tracking.
- Dataset Quality Assessment: Assess dataset quality, particularly label accuracy, to address discrepancies before engaging in a costlier modeling process to save cost and improve model metrics.
- Deeper Data Understanding: Deepen data understanding by identifying key phrases, unique terms, topics, and semantic clusters to establish or validate a hypothesis to frame a solution.
How MarkovML Enhanced Spectrum Labs' Machine Learning Workflow
Spectrum Labs tapped into the capabilities of MarkovML, achieving a significant transformation in data management, experimentation, and evaluation. By consolidating the following tasks on a single platform, Spectrum Labs not only simplified data discovery but also promoted efficient collaboration.

- Addressing Dataset Discrepancies: With MarkovML, Spectrum Labs gained new visibility into their datasets. The MarkovML platform revealed inconsistencies between training and testing sets, a revelation that held immense significance for real-world model applications.
By detecting these discrepancies early, Spectrum Labs ensured the accuracy and reliability of their content moderation models.
- Enhanced Data Understanding with Auto-EDA: MarkovML's Auto-EDA feature became Spectrum Labs' compass for navigating their data landscape. Through unsupervised algorithms, the platform uncovered vital phrases, topics, and clusters within the data.
This newfound understanding played a pivotal role in refining data labeling and recognizing intricate behavioral patterns that could have otherwise gone unnoticed.
- Interactive Visual Insights: One of MarkovML's standout features is its ability to present insights through interactive visualizations. Spectrum Labs harnessed this capability to enhance collaboration and stakeholder engagement.
Comparing results visually, drawing informed conclusions, and storing findings became effortless, leading to better alignment with diverse user needs.
- Tackling Labeling Inconsistencies: The accuracy of labeling directly impacts the efficacy of supervised machine learning. With MarkovML's Label Trust estimate, Spectrum Labs gained a comprehensive view of label quality.
This innovative tool enabled the team to promptly identify and rectify problematic records, thus enhancing the overall performance of their content moderation models.
- Tailored Model Evaluation: Spectrum Labs' clientele spans various sectors, each with distinct needs. MarkovML's model evaluation framework allowed for precise assessment of different model iterations, considering contextual nuances.
This flexibility empowered Spectrum Labs to customize solutions for individual clients, striking the delicate balance between various trade-offs.
- Automation: Before MarkovML, Spectrum Labs relied heavily on manual processes involving Python notebooks and Excel sheets. Tasks like exploratory data analysis, model evaluation, and results documentation were time-intensive.
With MarkovML, automation took center stage, significantly reducing manual effort. Drastically improving collaboration as insights became accessible without the need for deciphering complex code or spreadsheets.MarkovML emerged as the catalyst that streamlined Spectrum Labs' operations.
MarkovML's Value Realized
Before MarkovML, Spectrum Labs relied heavily on manual procedures using Python notebooks and Excel sheets. Routine tasks like exploratory data analysis (EDA), model evaluation, and results documentation consumed countless hours weekly.
But with MarkovML, much of this labor was automated, reducing manual interventions. Collaboration became more streamlined, with insights being easily accessible, all without the need to sift through complicated code or dense spreadsheets. MarkovML truly emerged as a catalyst for change at Spectrum Labs.
Let’s Talk About What MarkovML
Can Do for Your Business
Boost your Data to AI journey with MarkovML today!